The Coalition Against Insurance Fraud (CAIF)[1] estimated that acts of fraud cost US consumers at least $80 billion each year, with the workers’ compensation segment accounting for more than $30 billion of losses annually.
Despite rapid technological development, fraud in the insurance sector continues to escalate throughout the years. Whether it’s fraudulent claims, healthcare fraud, or identity theft, insurers face an uphill battle.
Hence, there is a huge need for fraud detection and prevention. In this blog, we will dive deep into the importance of implementing an automated fraud detection system and how Artificial Intelligence leverages the Insurance Industry.
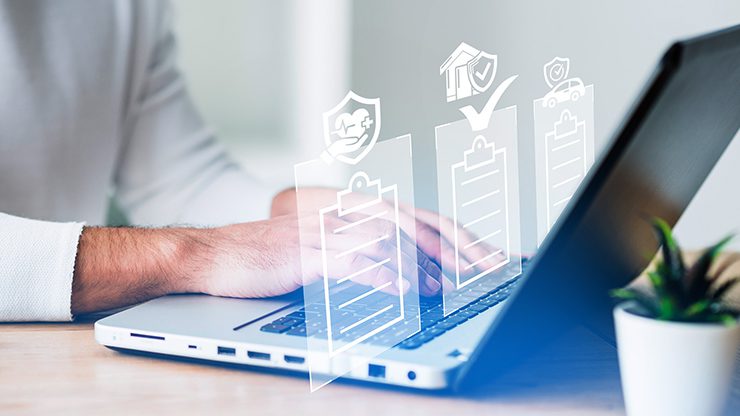
Cracking the Code: What is Fraud Detection Automation in the Insurance Industry?
Insurance is a binding contract between the insurance company and the insurer to protect an asset against uncertain risks.[2] In the insurance industry, fraud is one of the major challenges for insurance companies.
Insurance fraud may be committed by the policyholder or a third-party insurance policy claim[2]. Therefore, most insurance companies have devoted time and budget to fraud detection.
Fraud detection automation in the insurance industry refers to the use of technology and advanced analytical tools to identify and prevent fraudulent activities within the insurance sector.
Insurance fraud is a significant challenge for the industry, leading to financial losses and increased premiums for policyholders. Fraud detection automation aims to improve the efficiency and accuracy of identifying potentially fraudulent claims or activities.
From Manual to Digital: The Rising Demand for Automation
Old-school fraud detection methods rely on predefined rules and human analysis to identify fraudulent activity. These methods are often labor-intensive and cannot detect sophisticated fraud attempts. Other downsides of this method include:
- False positives: A rule that blocks all transactions from a risky region will prevent lots of genuine customers from approaching the business[3].
- Fixed outcomes: The thresholds for fraud behavior can change over time so rules can become invalid. Rules are also based on absolute yes/no answers, so don’t allow you to adjust the outcome or judge where a payment sits on the risk scale.
- Inefficient and hard-to-scale: Using a rules-only approach means that your library must keep expanding as fraud evolves. This makes the system slower and puts a heavy maintenance burden on your fraud analyst team.
Automated fraud detection by AI uses machine learning algorithms to analyze large amounts of data to identify anomalies that may indicate fraud. These algorithms learn from new data and adapt to changing fraud patterns.
Here is a table that summarizes the key differences between old-school fraud detection methods and automated fraud detection by AI:
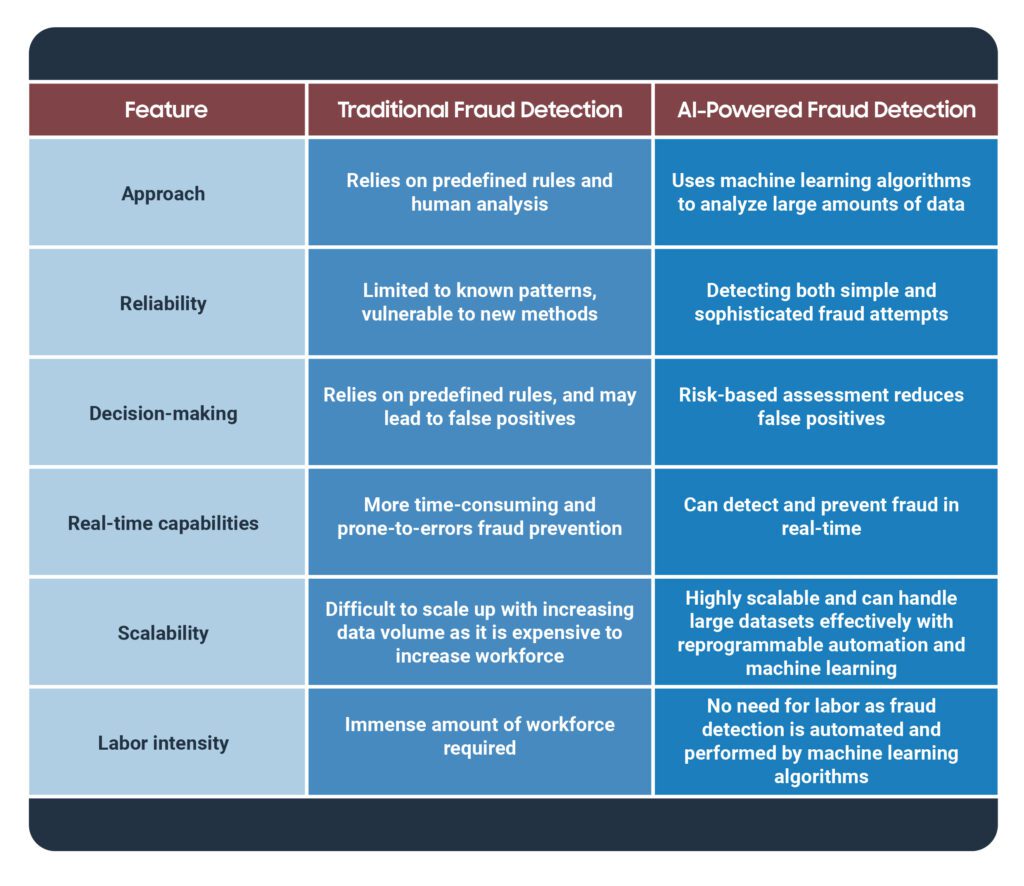
In traditional fraud detection, several repetitive manual tasks are time-consuming and expensive. Such steps include compliance checks, data entry, and verification, claims categorization, etc.
Automation in fraud detection alleviates the burden on human investigators significantly. They automate many of the tasks that are currently performed manually by fraud analysts, freeing up their time to focus on more complex investigations.
Overall, automated fraud detection by AI is a more effective and efficient way to detect fraud than old-school fraud detection methods. It provides a more comprehensive, adaptive, and scalable approach to fraud prevention.
As fraudsters continue to develop sophisticated techniques, AI will play an increasingly critical role in protecting businesses and consumers from financial losses.
Common Types of Insurance Fraud
Here are the most common types of fraud committed against various segments of the insurance sector, according to industry experts[4]:
- Automotive Insurance Fraud: This occurs when individuals intentionally cause accidents or exaggerate the damages to make fraudulent insurance claims.
- Health care fraud: This includes submitting false medical bills, overbilling, unnecessary medical procedures, or billing for services not rendered.
- Workers’ compensation fraud: This occurs when employers or employees make false claims or misrepresent injuries to receive workers’ compensation benefits.
- Disaster-related property fraud: Refers to intentionally setting fire to property or causing damage to claim insurance benefits.
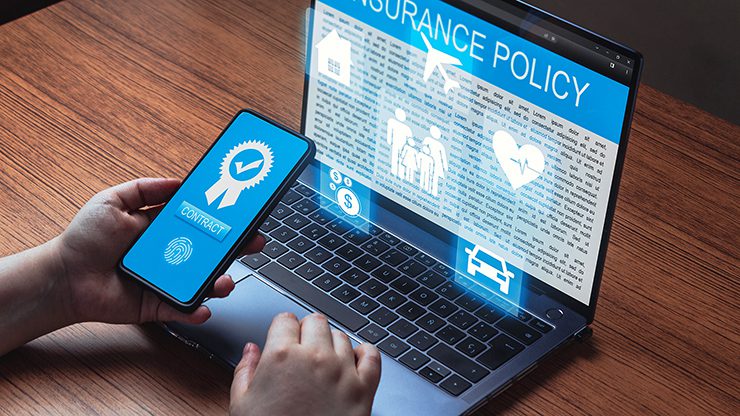
Benefits of Automated Fraud Detection Systems in the Insurance Industry
Automation is transforming the landscape of fraud detection. By automating various aspects of the fraud detection process, businesses can enhance their ability to identify and prevent fraudulent activities in a timely and effective manner.
Reduce Financial Loss
By reducing the number of fraudulent payouts thanks to fraud detection, insurance companies can maintain profitability. Additionally, automation streamlines the claims processing workflow, reducing operational costs and improving overall efficiency.
Preservation of Industry Reputation and Trust
By actively combating fraud and preventing fraudulent activities, insurance companies preserve the industry’s reputation, attract new customers, and retain existing ones. As a result, this leads to an increase in sales.
Regulatory Compliance
Fraud detection is essential for insurance companies to comply with regulatory requirements. By implementing robust fraud detection measures, insurance companies demonstrate their adherence to industry regulations and avoid penalties.
Improved Accuracy and Efficiency
Machine learning algorithms identify complex fraud schemes that may go unnoticed by traditional rule-based systems. By automating the process, insurance companies can improve fraud detection accuracy and minimize the risk of false positives or negatives[5].
Faster Claims Processing
Manual fraud detection processes can be time-consuming, leading to delays in claims processing and customer dissatisfaction. Once automated, fraud detection systems process genuine claims more quickly to improve customer satisfaction.
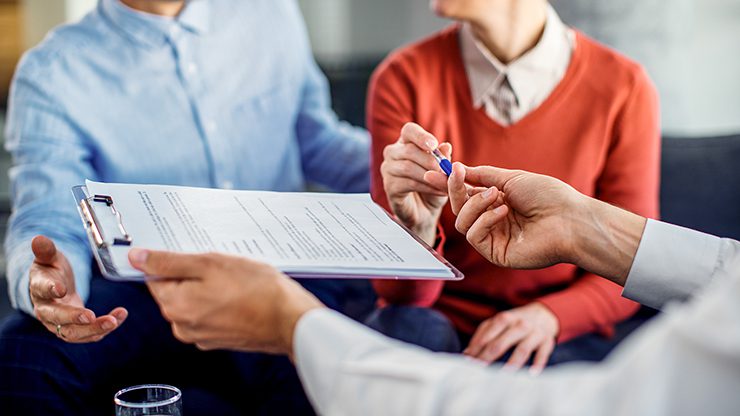
Enhanced Scalability and Adaptability
Automated systems easily scale to accommodate growing data volumes and adapt to evolving fraud patterns, ensuring that businesses are equipped to handle the ever-changing threat landscape.
Reduced Manual Labor
The workload of fraud investigators has been reduced since repetitive tasks have been automated. Hence, employees have more time to focus on more complicated tasks which should be prioritized to increase efficiency and productivity.
Detect, Defer, Defend: How AI Techniques Enhance Automated Insurance Fraud Detection
Artificial Intelligence (AI) automates the claims process to reduce fraudulent claims, monitor customer behavior, and identify abnormal activity that indicates fraud. Here’s how AI techniques employed in automation contribute to improved fraud detection:
Machine learning
Automatically identifies patterns of fraud in historical data. It helps detect fraud by analyzing large data sets to find patterns and anomalies. This data can then be used to train a model to flag potential fraud in future data.
Several types of Machine Learning Techniques Include[6]:
- Supervised learning: Uses a labeled dataset to train the model mapping input data to desired output labels. Once the model is trained with past data of known fraudulent claims, it can be used on future data to predict fraudulent activities.
- Unsupervised learning: Relies on the structure of the data itself to group claims according to amount, location, and type of claim. If there are groups that are significantly different from other groups, this could indicate fraud.
- Clustering: Groups data points that are similar to each other, and separates those that are dissimilar.
- Association Rules: This looks for relationships between items in data sets. For instance, people who file insurance claims for car accidents are also more likely to file claims for injuries.
- Anomaly detection: This looks for patterns in data that are unusual or unexpected such as claims that have unusually high payouts.
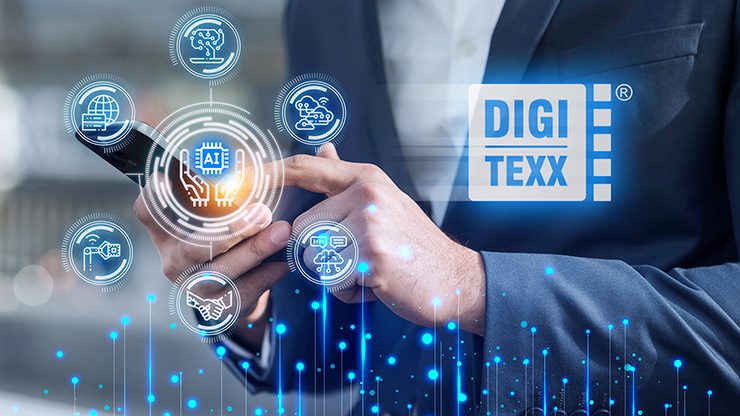
Deep learning is a type of Machine Learning that uses artificial neural networks (ANNs) inspired by the brain’s ability to learn. ANNs learn complex patterns in data and make predictions about new data points.
Natural language processing (NLP)
By extracting meaning from unstructured textual data such as claim descriptions and medical reports, NLP identifies potential fraud indicators that traditional rule-based systems may miss. It also analyzes customer reviews to detect negative indicators[7].
Computer Vision
In fraud detection, computer vision techniques examine visual evidence associated with insurance claims, such as accident scene photos or surveillance footage to identify inconsistencies or signs of manipulation, flagging potentially fraudulent claims[8].
The Future is Now: Real-Life Applications of Automated Fraud Detection for Insurance Claims
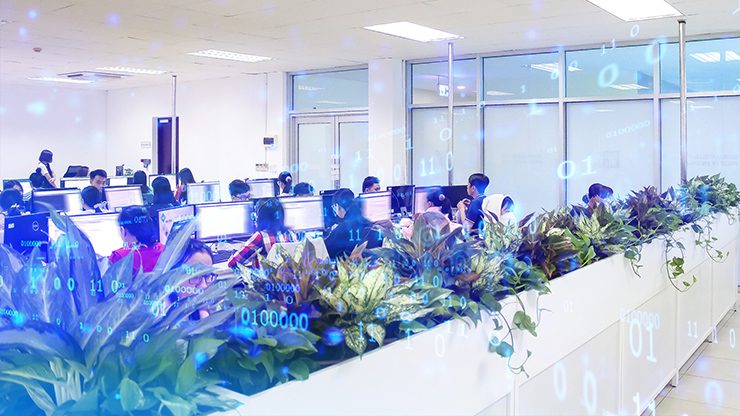
- Customer Onboarding Processes
According to the Customer Experience Officer[9] at Edelweiss Tokio Life Insurance, their customer onboarding processes have been streamlined by implementing several AI techniques:
- Anomaly Detection: ML analyzes the speed at which a customer fills out forms, the sequence of actions taken, or the device used for the transaction. Sudden deviations from typical patterns can trigger alerts for further investigation.
- Natural Language Processing (NLP): Analyze the content and language used in customer-provided text, such as application forms or communication messages, to identify potential fraudulent intent in the information provided[10].
- Car Policy Insurance
The Director of Engineering at Bimaplan[9] stated that automation facilitates instant policy issuance and real-time claim settlement. AI techniques implemented include:
- Computer Vision in Visual AI takes photos of a damaged vehicle and assesses the claim’s value based on what it knows about that car, and the extent of the damage, and estimates repair costs[11].
- Machine Learning (ML) techniques such as clustering and anomaly detection are integrated to make the system more intelligent to classify an insurance claim as a fraud or legitimate[12].
Uncovering behavior patterns such as requiring injury claims that do not match with the reports of the incidents helps companies prevent fraudulent activity without the need for human intervention.
Thus, automation plays an indispensable role in detecting fraud within the Insurance Industry.
From Deception to Detection: Let Automation Prevents Fraud and Safeguards Insurance Claims!
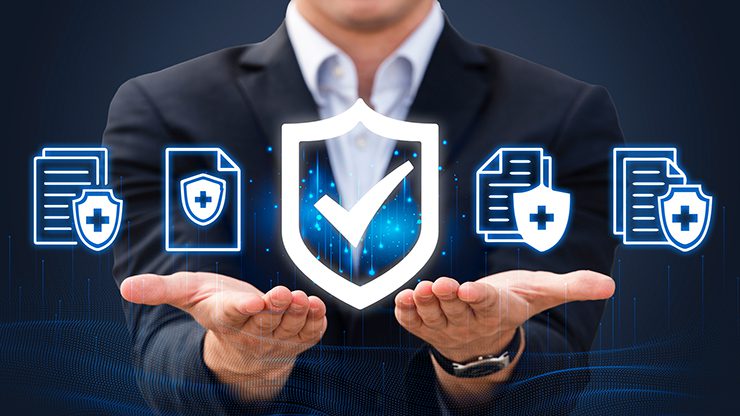
Automated fraud detection is crucial for safeguarding the insurance industry from the increasing threat of fraudulent activities.
By leveraging advanced technologies such as AI, ML, and NLP, insurance companies enhance their fraud detection capabilities and maintain a competitive edge while ensuring increased customer satisfaction and trust.
Fraud Detection through Automation for Insurance claims improves efficiency, lowers overhead costs, and enables insurance companies to stay ahead of fraudsters.
As the insurance industry continues to evolve, embracing automation in fraud detection will be instrumental in safeguarding its future.