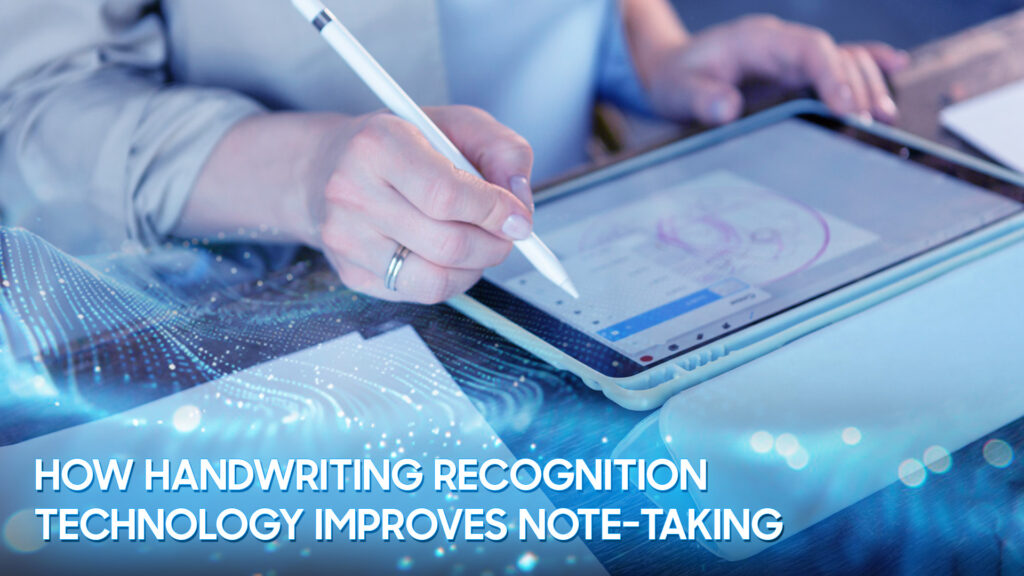
What is Handwriting Recognition Technology?
Handwriting recognition is a technology that enables computers or other devices to interpret and convert handwritten text into digital, machine-readable formats. It involves the use of algorithms and machine learning techniques to analyze the shapes, patterns, and strokes of handwritten characters, words, or symbols and translate them into typed text or commands.
There are two main types of handwriting recognition:
- Offline Handwriting Recognition: This involves analyzing static, pre-written text, such as scanned documents or images of handwriting. The system processes the visual data after it has been created, without real-time input.
- Online Handwriting Recognition: This occurs in real-time as the user writes, typically on a touchscreen device like a tablet or smartphone using a stylus or finger. The system captures the dynamic data of the writing process, such as stroke order, speed, and pressure, to improve accuracy.
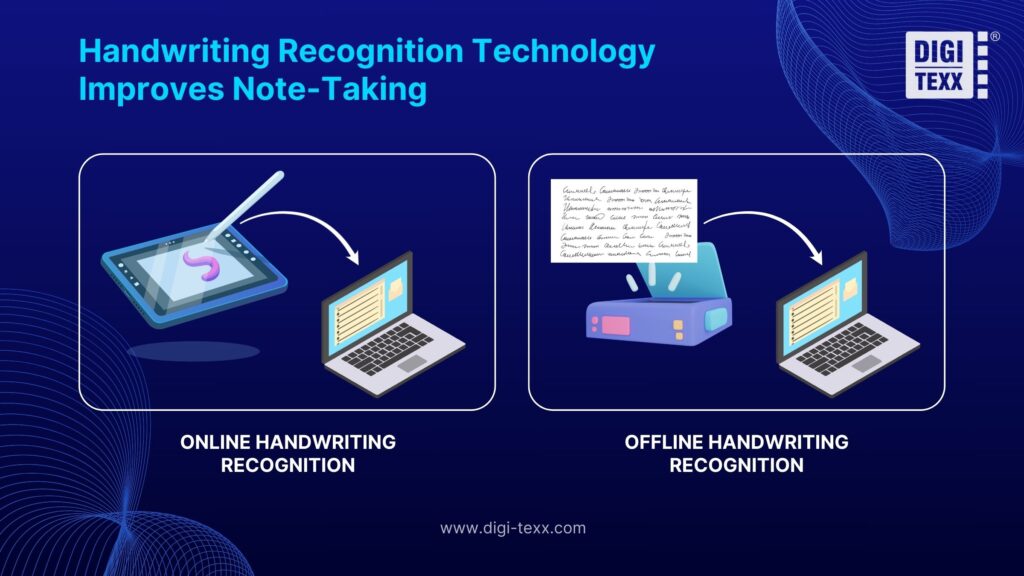
Handwriting recognition relies on techniques like optical character recognition (OCR) for offline systems and pattern recognition for online systems. It’s widely used in applications such as digitizing historical documents, processing forms, enabling stylus-based note-taking apps, and assisting people with disabilities by converting their handwriting into text.
The accuracy of handwriting recognition depends on factors like the clarity of the writing, the complexity of the script, and the sophistication of the underlying software. Modern systems often use artificial intelligence, including neural networks, to adapt to individual handwriting styles and improve over time.
Benefits of Handwriting Recognition Technology
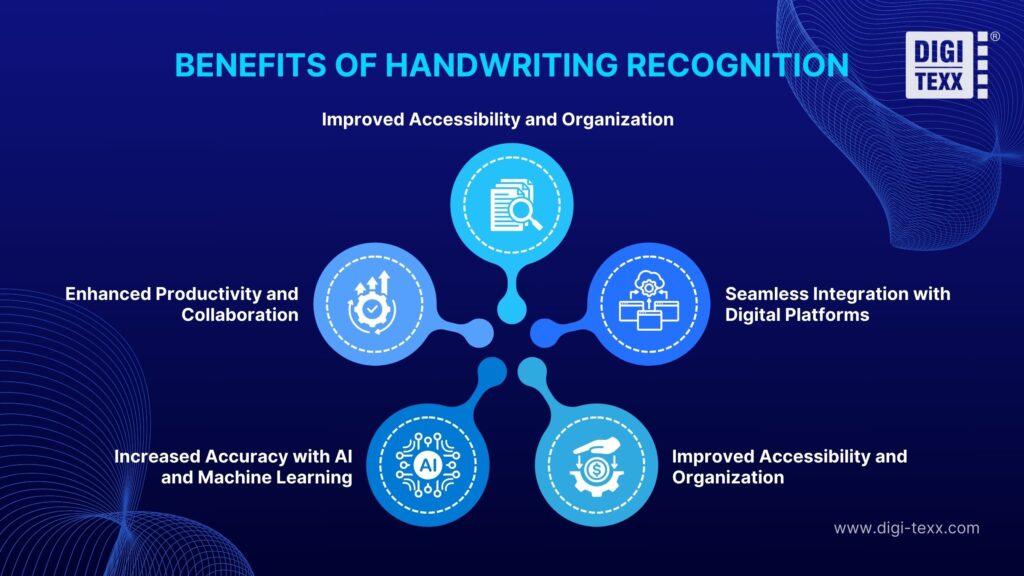
1. Improved Accessibility and Organization
Handwritten notes can be difficult to organize and retrieve due to their unstructured format. Unlike digital notes, they cannot be easily searched or backed up, increasing the risk of losing important information. Over time, managing piles of physical notes becomes inefficient and time-consuming.
Handwriting recognition technology solves this challenge by converting handwritten content into structured digital text, allowing for seamless storage, categorization, and keyword-based search. This is especially valuable for professionals who require quick and reliable access to critical information.
2. Enhanced Productivity and Collaboration
Handwriting recognition (HWR) technology revolutionizes digital note-taking by removing the need for manual transcription, significantly reducing the time and effort required to convert handwritten content into a usable format. Instead of retyping notes or struggling to decipher messy handwriting, users can instantly digitize their notes with accuracy.
Beyond efficiency, digitized notes offer seamless sharing across teams and departments, enhancing collaboration and ensuring that valuable insights are easily accessible to everyone. This is particularly useful in corporate environments where streamlined communication and knowledge management are essential.
3. Seamless Integration with Digital Platforms
HWR technology is built to work smoothly with various digital platforms, including cloud storage, project management software, and CRM systems. This allows users to convert handwritten notes into digital text that can be easily stored, organized, and accessed across multiple devices.
With this integration, businesses can streamline workflows by ensuring that important information is always available, reducing the risk of misplaced notes and improving overall efficiency in data management and collaboration.
4. Increased Accuracy with AI and Machine Learning
Modern handwriting recognition systems are designed to get better over time by learning from large amounts of data. These systems use artificial intelligence (AI) to understand not just the individual characters but also the context in which they appear.
They can identify the meaning behind the text, fix mistakes, and adjust to different handwriting styles. As a result, they become increasingly accurate and reliable, making them ideal for use in professional and business settings where precision and efficiency are crucial.
5. Eco-Friendly and Cost-Effective Solution
Switching from using paper to digital formats for taking notes helps reduce the amount of paper used and lowers printing costs. This shift supports environmental goals by cutting down on waste. For businesses and schools, it’s a cost-effective way to save money while also being more eco-friendly in how they manage information.
Challenges of Handwriting Recognition Technology
Handwriting recognition technology has come a long way, but it still faces several challenges that limit its efficiency and accuracy. Here are some of the key obstacles:
1. Variation in Handwriting Styles
One of the biggest challenges with handwriting recognition technology is the wide variety of handwriting styles.
Each person has a unique way of writing, from the shapes of characters and stroke connections to slant and spacing. This makes it difficult for the system to recognize characters accurately.
The issue becomes even more complicated when dealing with cursive or irregular handwriting, where characters may be connected or vary in shape compared to the standard.
Additionally, abbreviations and the use of non-standard writing conventions pose a significant barrier. Many individuals develop their own shorthand or use informal language conventions, such as teencode, personal symbols, or regional slang, which makes recognition more challenging.
Moreover, the difference in handwriting styles between countries and cultures also adds complexity to the system.
Examples of Popular Handwriting Styles:
- Fraktur (Germany): A Gothic-style script often seen in historical documents.
- Spencerian (USA): A delicate, cursive style commonly used in correspondence and administrative documents.
- Copperplate (UK): A round, consistent handwriting with a high slant, commonly used in calligraphy.
- Kaisho (Japan): A clear, standardized handwriting style resembling print, often taught in basic education.
- Thư pháp (Vietnamese): Known for its graceful, flowing strokes, used in artistic works like calligraphy paintings.
- Shufa (Chinese): Characterized by bold, sharp strokes, often used in artistic works and traditional calligraphy.
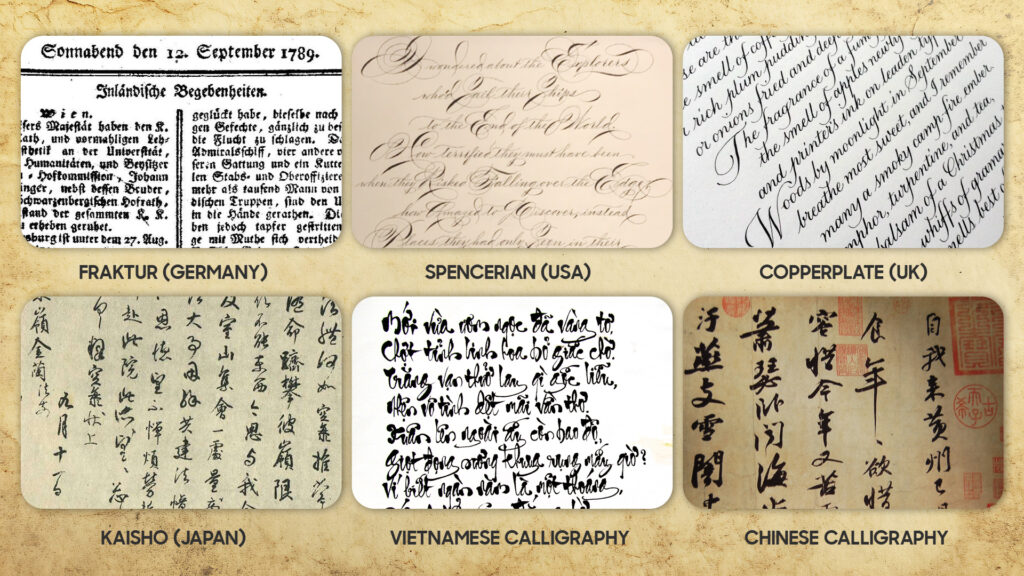
2. Ambiguity and Inconsistencies
Handwritten letters can often be ambiguous, especially when they are poorly formed or when multiple letters appear similar.
For instance, the uppercase “L” and lowercase “l” can be hard to distinguish, particularly when the lowercase “l” is written with a stroke similar to a dash or a simple line.
This can lead to misinterpretation and errors, requiring advanced algorithms to resolve inconsistencies and correctly identify characters.
Such challenges highlight the complexity of handwriting recognition technology, which must be able to accurately differentiate even subtle variations in handwriting styles.
3. Noise and Distortions in the Data
Scanned or photographed handwriting often comes with imperfections that can affect the recognition process. For example, noise and distortion from scanning or low resolution can blur or obscure the text, making it harder for the system to accurately identify the characters.
Additionally, uneven pen pressure or ink smudging can cause certain letters or words to appear unclear or inconsistent. These imperfections introduce errors, requiring advanced algorithms and image processing techniques to clean up the data and ensure accurate recognition.
4. Language and Context Sensitivity
Handwriting recognition systems often face difficulties when handling different languages or symbols, especially when the writing includes a mix of languages. Each language has its own set of characters and grammar rules, which can confuse the system.
For instance, cursive writing or special characters unique to certain languages might not be easily recognized. Furthermore, recognizing context-specific variations such as names, technical terms, or abbreviations can be challenging, as these may not follow standard writing patterns or may have unconventional spellings, making it harder for the system to interpret them accurately.
5. Limited Training Data
Handwriting recognition algorithms need to be trained on a large volume of high-quality data to enhance their accuracy. The more diverse the handwriting styles included in the training data, the better the system can recognize various types of writing.
However, collecting this data can be challenging. It requires a substantial amount of time and effort to gather samples from various people, especially with different handwriting styles, and ensure the data is of high quality. This process can be both time-consuming and resource-intensive, as it involves careful labeling and curating of data to ensure the system learns effectively.
6. Real-Time Processing
Real-time handwriting recognition, particularly on mobile devices or with large amounts of data, can be quite challenging. Processing handwriting input quickly and accurately without causing noticeable delays is essential for a smooth user experience.
However, achieving this consistently is difficult due to the varying quality of input, device limitations, and the need for immediate recognition. The system must be able to process data in real-time while maintaining accuracy, which becomes more complex when dealing with large volumes of handwriting or less powerful devices. This requires advanced algorithms and optimizations to ensure the technology remains responsive and efficient.
7. Integration with Other Systems
Many handwriting recognition solutions are still standalone, meaning they work independently without easily connecting to other applications or databases. This lack of integration can make it difficult to incorporate recognized handwriting data into broader workflows or systems.
Ensuring smooth communication between handwriting recognition tools and other software, such as databases, customer management systems, or document processing applications, can be complex.
Without seamless integration, data may need to be manually transferred or reformatted, adding time and effort to the process. This ongoing challenge highlights the need for better interoperability across digital platforms to maximize the efficiency and value of handwriting recognition technology.
8. Recognition of Non-Alphanumeric Characters
Non-alphanumeric characters, such as punctuation marks, mathematical symbols, and special characters, can be particularly challenging for handwriting recognition technologies. Unlike letters and numbers, which tend to follow more consistent shapes and patterns, these characters vary widely in form and are often less standardized in handwriting.
For example, a comma or period might be written in different ways depending on the writer’s style, making it difficult for the system to recognize them reliably. This inconsistency increases the likelihood of errors in recognizing non-alphanumeric characters and requires more advanced algorithms to interpret them accurately.
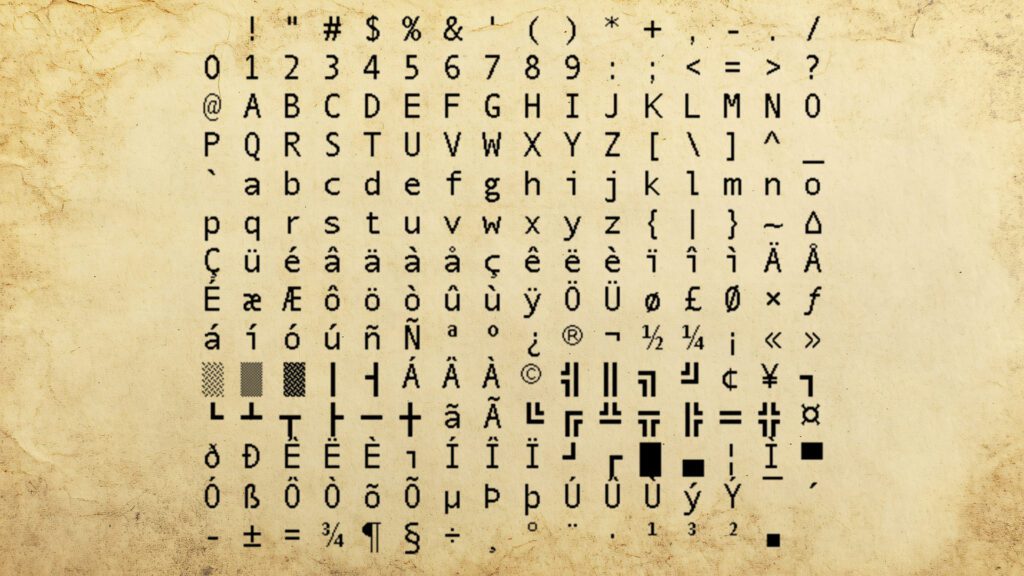
Methods of Handwriting Recognition
Handwriting recognition technology utilizes various methods to convert handwritten text into digital formats. These methods can be broadly classified into offline and online recognition systems.
Method | Description | Advantages | Disadvantages |
Manual | Humans manually read, analyze, and input handwritten content. | High accuracy, effective with unclear handwriting. | Time-consuming, labor-intensive, high operational cost. |
Automated | Software systems automatically recognize and digitize handwriting. | Fast processing speed, cost-effective long-term. | Lower accuracy with illegible or non-standard handwriting. |
Hybrid | Combines automated recognition with human review and correction. | Balanced accuracy and efficiency. | Still requires human resources, slower than full automation. |
Handwriting Recognition Techniques
Handwriting recognition is the process of translating handwritten text into machine-readable data. This technology has evolved significantly over time, and various techniques are employed to recognize handwriting effectively. Here are some of the key techniques used in handwriting recognition:
Technique | Description | Data Type | Technique Type | Application |
1. Preprocessing | ||||
OpenCV / PIL | Enhance image quality, remove noise, and binarize | Image | Image Processing | Prepare input image for recognition |
Skimage | Image transformation, morphological operations | Image | Image Processing | Line/character isolation, contrast adjustment |
Skimage | Image transformation, morphological operations | Image | Image Processing | Line/character isolation, contrast adjustment |
Tesseract Layout Analyzer | Detect layout structure, segment lines and words | Image (Document layout) | Layout Analysis | Line and paragraph segmentation |
2. Feature Extraction | ||||
HOG (Histogram of Oriented Gradients) | Extract edges, stroke direction, local shape features | Image patches, grayscale images | Feature-based | Shape/character analysis |
SIFT / SURF | Detect and describe local keypoints | Grayscale image | Feature-based | Character recognition, template matching |
CNN Layers | Automatically extract spatial patterns and features | Raw image | Deep Learning | Input to classification models |
3. Matching | ||||
Template Matching | Compare handwriting to predefined templates | Image or vector strokes | Pattern Matching | Simple handwriting recognition tasks |
Dynamic Time Warping (DTW) | Match time-series handwriting strokes for alignment | Sequential stroke data | Time-series Pattern Matching | Signature verification, handwriting comparison |
4. Classification | ||||
OCR Engines (Tesseract) | Recognize characters from segmented text regions | Processed image | Machine Learning + Rule-based | Document OCR, form digitization |
K-Nearest Neighbors (KNN) | Classify based on similarity to training samples | Vectorized features | Supervised Learning | Character recognition in structured forms |
SVM (Support Vector Machine) | Find decision boundaries for character classification | Extracted features | Supervised Learning | Image/character classification |
CNN (Convolutional NN) | Learn and classify characters via deep image features | Raw or preprocessed image | Deep Learning | Real-time handwriting recognition |
RNN / LSTM | Sequence modeling, capture handwriting flow and context | Sequential image or stroke data | Deep Learning | Handwriting-to-text conversion |
HMM (Hidden Markov Model) | Model sequential character transitions probabilistically | Sequential image or features | Probabilistic Modeling | Handwriting line recognition, speech-to-text |
5. Post-processing | ||||
Spell Checkers (e.g., Hunspell) | Correct errors in recognized text using dictionaries | Text | Rule-based / NLP | Improve textual accuracy |
Language Models (n-gram, BERT) | Use context to refine and predict correct text | Text | Statistical / Neural Language Model | Improve sentence coherence, reduce misrecognition |
Human-in-the-loop Review | Manual verification and correction of output | Text + image overlay | Manual / Hybrid Workflow | Quality assurance for critical applications |
Real-World Applications of Handwriting Recognition Technology in Business
Handwriting recognition technology is transforming how businesses handle handwritten documents, improving efficiency, accuracy, and data accessibility. Below are key industries where this technology is making a significant impact:
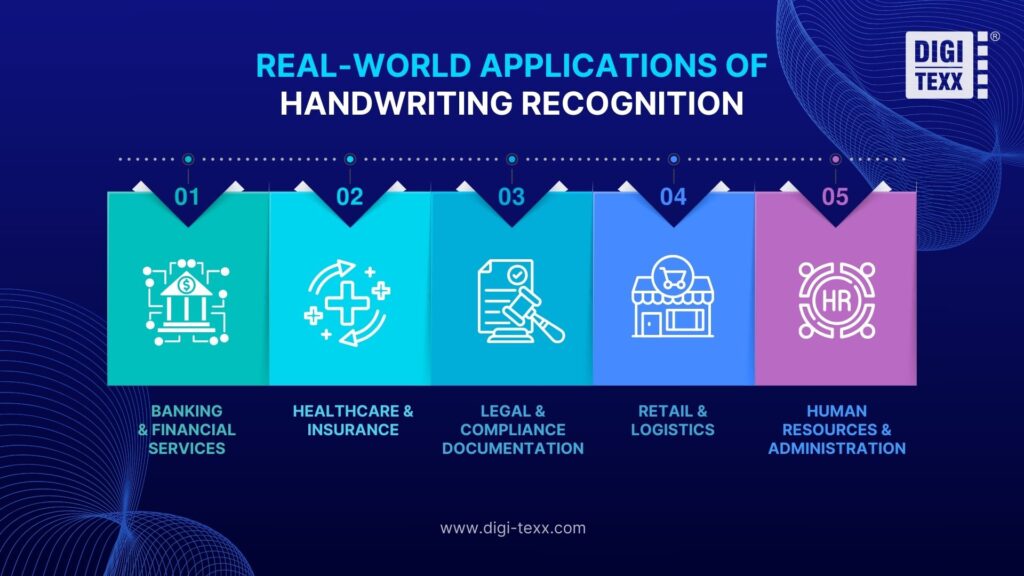
1. Banking & Financial Services
In banking, many processes still involve handwritten documents, such as checks, loan applications, and customer forms. Handwriting recognition automates these tasks by extracting data from these documents, reducing the need for manual entry.
- Automated Check Processing:
Instead of manually verifying checks, banks use handwriting recognition to scan and authenticate the information on checks. This process reduces human error and speeds up transaction processing, saving time and improving efficiency.
- Loan & Account Form Digitization:
When customers fill out handwritten forms for loan applications or account openings, handwriting recognition technology automatically converts the handwritten data into a digital format. This ensures accuracy and enables fast and efficient data processing, eliminating errors that can occur with manual data entry.
- Signature Verification:
Banks and financial institutions use handwriting recognition technology to authenticate signatures on contracts and financial transactions. This helps mitigate the risk of fraud, as it can compare the actual signature with a stored reference to ensure validity.
2. Healthcare & Insurance
The healthcare and insurance industries manage a significant volume of handwritten documents, ranging from patient records to claim forms. Handwriting recognition technology simplifies the extraction and storage of this data, enhancing service quality and ensuring better compliance.
- Electronic Medical Records (EMR):
Doctors and medical staff often take handwritten notes during patient consultations. Handwriting recognition converts these notes into digital records, making them easily accessible and reducing the need for paper-based documentation.
- Claims Processing Automation:
Insurance companies receive a large number of handwritten claim forms. Rather than manually entering information, handwriting recognition technology extracts key details, accelerating the approval process and reducing processing costs.
3. Legal & Compliance Documentation
Law firms and compliance departments handle a wide range of handwritten documents, such as contracts, agreements, and regulatory reports. Handwriting recognition ensures these records are digitized, improving organization and ensuring legal compliance.
- Contract & Agreement Management:
Rather than manually transcribing handwritten contracts, law firms can use handwriting recognition to digitize them, making retrieval and review faster and more efficient.
- Regulatory Compliance:
Businesses are required to submit various compliance documents. Handwriting recognition helps digitize and archive these reports, minimizing the risk of document loss or misinterpretation.
4. Retail & Logistics
Retailers and logistics companies still rely on handwritten order forms, invoices, and delivery receipts. Digitizing these documents enhances tracking, customer service, and operational efficiency.
- Order & Delivery Processing:
Couriers and logistics providers manage thousands of handwritten delivery forms each day. Handwriting recognition converts these forms into digital records, improving tracking accuracy and operational efficiency.
- Customer Feedback & Surveys:
Retailers collect customer opinions through handwritten surveys. With handwriting recognition, these responses can be quickly digitized and analyzed, providing valuable insights for better decision-making.
5. Human Resources & Administration
HR and administrative teams handle a high volume of employee-related paperwork, including job applications, onboarding documents, and meeting notes.
- Employee Records & Onboarding Forms:
In HR departments, many documents, such as job applications, employee records, and onboarding forms, are still handwritten. Handwriting recognition technology converts these documents into digital data, making them easier to store, access, and manage. This not only saves time but also minimizes errors that can occur with manual data entry, ensuring that the information is organized more efficiently.
- Meeting Notes & Internal Documentation:
Meetings often involve handwritten notes taken by employees and executives. Handwriting recognition transforms these notes into searchable digital text, making collaboration easier and enabling quicker reference to important information. This is especially helpful when the notes need to be revisited or shared with others in the organization.
Handwriting Recognition Technology: Boosting Efficiency and Accuracy for Your Business
Handwriting recognition technology is revolutionizing how businesses handle handwritten data by digitizing documents and automating data entry. This reduces errors, saves time, and allows employees to focus on more valuable tasks. Whether for invoice processing, form filling, or customer feedback, handwriting recognition ensures fast and accurate data capture.
For businesses in industries like healthcare, banking, or legal services, where accuracy and data security are critical, adopting this technology can lead to streamlined operations, better decision-making, and improved customer service. As AI continues to advance, the potential of handwriting recognition technology will only expand, making it an essential tool for businesses seeking to stay ahead of the curve.
Now is the time for your business to leverage handwriting recognition technology to unlock new levels of efficiency and enhance data accuracy, ultimately driving better outcomes.