Automated document processing is an essential need for modern businesses that are overloaded with document processing tasks. Manual processing tasks are often inefficient and prone to errors. In this article, DIGI-TEXX will explain the terms of automated document processing – the key to achieving efficiency and competitive advantage in today’s data-centric world.
=> Read more: Choosing the Right Document Processing Company for Your Needs
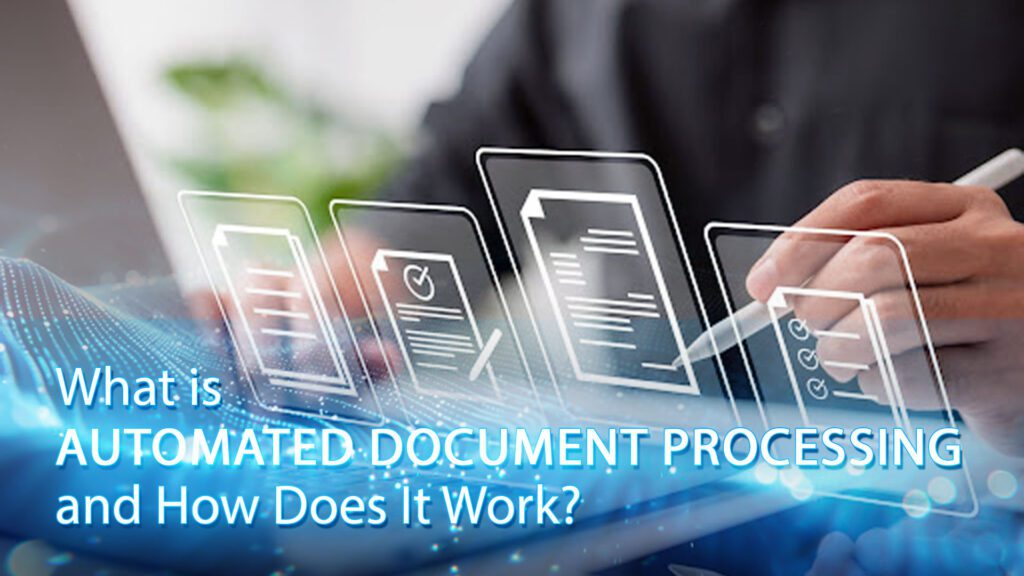
What is Automated Document Processing?
Automated document processing (ADP) is the use of software technologies and algorithms, especially artificial intelligence, to automatically collect, classify, extract, authenticate and integrate data from various document sources.
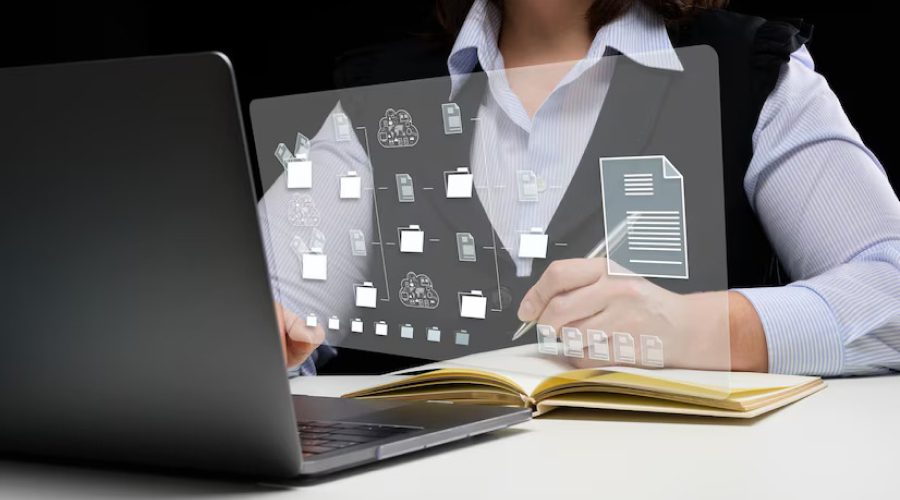
These document sources can be digital files such as PDFs, scanned images, emails and possibly even digitized paper documents. The main goal of automated document processing is to convert the information stored in these documents, which are often unstructured or semi-structured, into structured, machine-readable data formats that enable systems to forecast and make business decisions. Automated document processing unlike traditional manual methods, which are error-prone and inefficient when processing large volumes of data.
The technologies used in automated document processing are derived from the integration of various technologies. In essence, ADP combines AI, Machine Learning (ML), Deep Learning (DL), Natural Language Processing (NLP), and Computer Vision. AI and ML algorithms allow systems to learn from data, recognize patterns, and improve performance over time without the need for extensive programming.
NLP will help software understand, interpret, and even generate human language contained in documents, capturing their context and meaning. Computer vision enables systems to ‘see’ and interpret images and document layouts. This combination of technologies is important because it empowers ADP systems to process not only highly structured documents (like spreadsheets) but also common semi-structured documents: like invoices, contracts, emails or reports.
=> See more: Understanding Natural Language Processing: A Comprehensive Guide
This capability represents a significant step forward from legacy technologies like basic Optical Character Recognition (OCR), which primarily focused on converting images of typed text into machine-readable text. ADP is therefore more than just a technology; the process represents a strategic capability for intelligent data processing, simulating the environment for document interpretation.
=> You might like: Choosing the Right Data Processing Services for Your Needs
The key strength of automated document processing is its ability to manage a wide variety of document types and formats, regardless of their volume or complexity. Businesses will regularly process invoices, purchase orders, contracts, human resources forms, identification documents (ID cards, passports, birth certificates), insurance claims, medical records, financial statements, etc.
ADP solutions are specifically designed to handle these types of documents, whether they are in PDF, JPEG, TIFF, email, etc. Furthermore, the solution and technology are built to be scalable, capable of handling fluctuations in document volume, from hundreds to millions of pages per month, without affecting overall performance.
=> See more: Top Solutions in Automated Document Processing Software
How does document processing automation work?
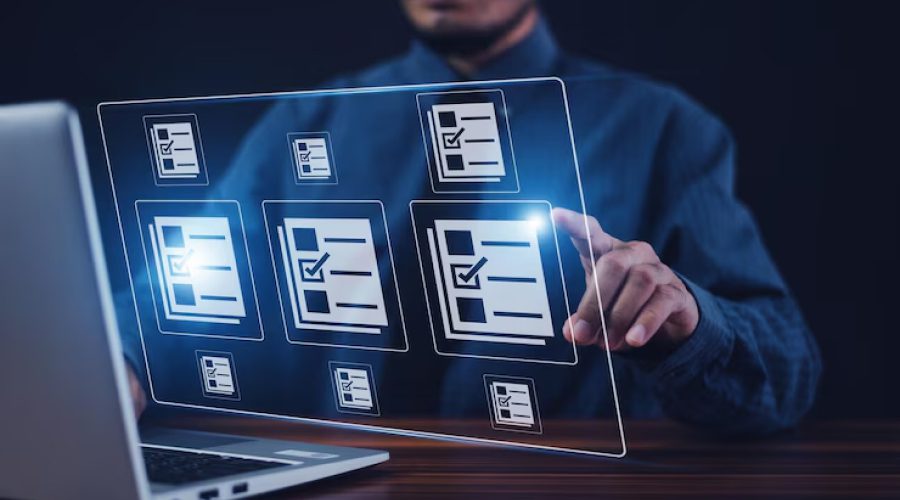
Document processing automation will operate based on a structured workflow, which will have multiple stages designed to transform raw document data into organized information that can be easily integrated into business forecasting systems. While the actual implementation will vary from case to case, the core process typically includes basic steps, ensuring a logical progression from document capture to data utilization. A good document processing automation process relies heavily on the efficiency and accuracy of each interconnected stage.
Collect/Ingest
The process will begin with the capture of documents into the ADP system. This stage will have a variety of input methods to suit different needs. Physical documents will typically be scanned, creating digital images. Digital files, such as PDFs, Word documents, or image files (JPEG, PNG, TIFF), can be uploaded or imported directly. If the process has integration capabilities, it will be possible to automatically retrieve documents from email inboxes, cloud storage platforms (such as Dropbox or SharePoint), ERP systems, or APIs.
In addition, this step will be supported by image processing algorithms, including noise reduction (removing background spots or marks), skewing (correcting skewed images), cropping irrelevant areas, and adjusting brightness and contrast to make the text clearer and more readable for subsequent OCR processing. High-quality input at this stage is essential for high accuracy in document process automation.
Classification
After collection and pre-processing, the system must understand what type of document it is processing. Document classification will automatically use AI technologies, including ML, NLP, and Computer Vision, to analyze the content, structure, layout, and repeating patterns of the document. Some systems will use techniques such as vectorization to represent the document in digital form for classification. Accurate classification is important because it is the foundation for determining the extraction rules, authenticity checks, and specific processing procedures to be applied to the document in the following steps. Incorrect classification can lead to incorrect data extraction or processing errors in the automated document processing process.
Extraction
Optical Character Recognition (OCR) technology will be used in this step to convert the visual representation of text (from scans or images) into machine-readable character data. For handwritten text, more advanced Intelligent Character Recognition (ICR) can be used for processing. However, text transformation alone is not enough, especially for semi-structured and unstructured documents.
This is where AI, ML and NLP techniques come into play. Technologies like Named Entity Recognition (NER) will identify and extract specific data points – such as supplier name, invoice number, date, total amount, line item details, customer address, contract terms or specific terms – based on context and learned patterns for analysis. Advanced technologies will help with context awareness for different variable formats, sometimes involving field detection based on labels or locations containing information.
Validation
The extracted data will not be usable initially; therefore, the process will require a validation step. The system will have to validate the extracted information for accuracy, completeness, and consistency using various techniques. Such as cross-referencing the data with an external or internal database, comparing the supplier details on the invoice with data from the primary supplier, or matching against the purchase order data (two-way or three-way matching). At this point, the system can detect duplicates or data errors (data points that appear unusual or are misclassified).
Data enrichment can also occur at this step, where missing information is added or existing data verified by external sources is added to make the data complete. Many systems can measure confidence scores for the extracted information fields to visually represent this step. When anomalies arise, processing personnel can review the flagged data, correct errors if necessary, and re-validate the data if necessary. This not only ensures the accuracy of critical data, but also provides valuable feedback to ML models, helping them learn and improve over time.
Integration
During document processing automation, once validation is complete, the data is mapped to the company or organization’s data repository. The processed data is converted into formats such as JSON, XML, CSV, XLSX, or text. This structured data is then mapped to ERP systems, CRM systems, accounting software (for bookkeeping), databases, data warehouses (for analytics), or other workflow automation tools. This integration will be through APIs or middleware platforms, ensuring a smooth data flow and enabling other systems to act on the extracted information. This final step closes the loop, transforming static documents into dynamic data that drives business processes.
Benefits of Using Document Automation Processes
Implementing document automation processes has strategic benefits: helping the bottom line and operational efficiency of the organization.
Increased efficiency and productivity in document processing
Document automation processes automate repetitive, time-consuming manual tasks such as data entry, document classification, storage, and retrieval. When these activities can be automated, businesses can reduce document processing time from hours or days to minutes. This acceleration eliminates bottlenecks in workflows and ensures that information flows smoothly throughout the organization and company. This significantly boosts employee productivity, redirecting their time and expertise to more strategic, analytical, and customer-oriented activities
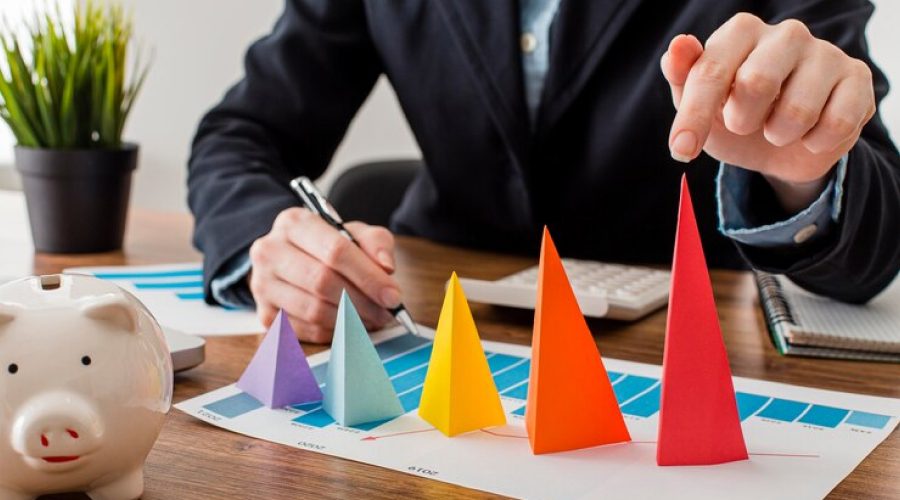
Enhanced data accuracy and consistency
Manual data entry is prone to errors due to human intervention – typos, misunderstandings, and omissions are common. Document automation processes significantly reduce these errors by minimizing manual intervention. Advanced ADP systems, leveraging AI and automated validation checks, can achieve high accuracy rates when extracting data. With the automated validation step rigorously checking data, organizations and companies can ensure that documents are processed and data is collected in a systematic manner, significantly contributing to improving the quality of information for companies and organizations.
Improved data security and access control measures
Digitizing documents through automated process documentation also inherently supports increased security within the organization. Digital data can be protected by encryption, both in transit and at rest. Document automation process facilitates the implementation of job-specific access controls, ensuring that only authorized employees can view or modify specific documents or data fields based on their job function. Systems can record logs, recording who accessed which documents, when, and what changes were made. Compliance with regulations such as GDPR or HIPAA is ensured.
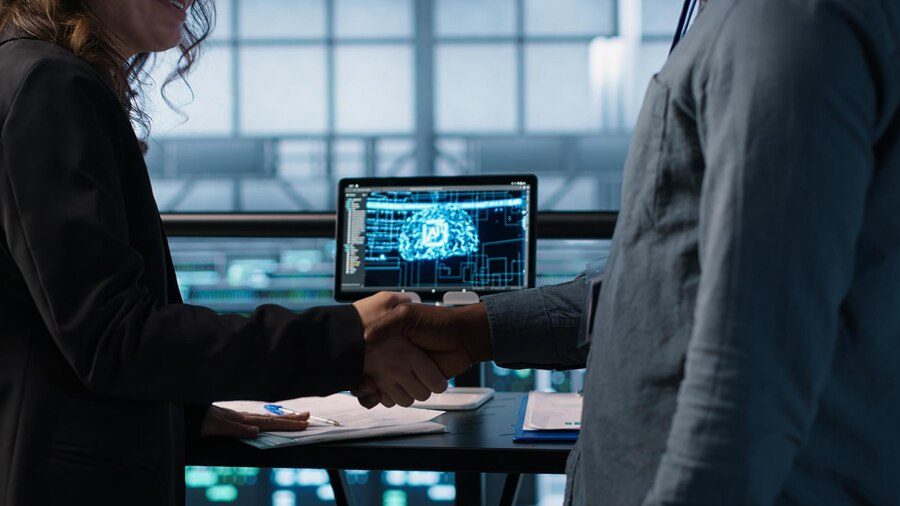
Cost savings and reduced paper usage
Companies can significantly reduce costs associated with paper, printing, ink, and physical storage space. Automating manual tasks can save significant personnel costs. Some businesses report saving thousands of dollars per month or significantly reducing staff costs. Reduced error rates will also save costs associated with correcting errors, paying late fees, or dealing with contract penalties. Additionally, moving to digital processing will also promote a paperless or “paperless” office environment.
Faster decision-making with real-time data access
In a world where every decision is driven by data, having accurate information is critical to effective decision-making. Document automation processes facilitate this by converting static documents into digital data for analysis. Because documents are processed much faster and stored digitally, authorized users can access critical information anytime, anywhere. Furthermore, structured data generated by ADP systems can be fed directly into business analytics platforms, providing real-time insights into operational performance, customer behavior, and market trends, further enhancing strategic decision-making.
=> See more: How Real-Time Data Scraping Transforms Data Collection
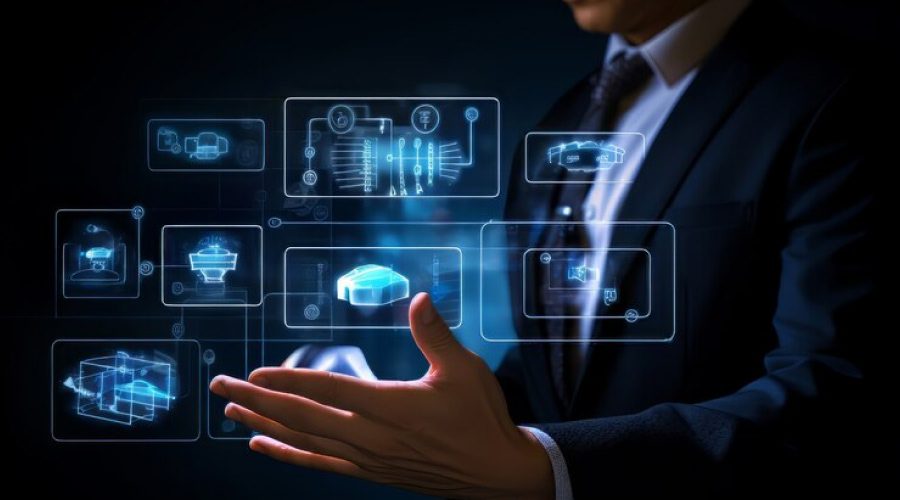
Best Tools for Document Automation Processing
The document automation solutions processing market is diverse, offering a wide range of tools with different capabilities, strengths, and target audiences. Choosing the right tool is crucial and depends largely on the specific requirements of the organization or company. In the current market, here are five prominent tools that can be referred to
Experlogix Document Automation
Positioned as an easy-to-use, low-code, AI-powered solution, Experlogix is suitable for companies that handle highly complex document workflows. The tool emphasizes self-use, allowing users without deep technical skills to design and manage custom document processes. Key strengths include seamless integration with major CRM and ERP systems such as Microsoft Dynamics, leveraging existing company data for implementation. Additionally, Experlogix features enterprise content management (ECM) for security and is known for its flexibility and competitive pricing in the market.
Quadient Inspire
Quadient Inspire excels at automating multi-channel customer communication management (CCM), making it an ideal choice for complex document creation and distribution, such as for insurance, government, and financial services organizations. Quadient Inspire has an intuitive drag-and-drop interface for designing documents and workflows. While it is AI-powered and low-code friendly, its cloud storage capabilities are limited and additional paid modules are always required for full functionality, which can increase the overall cost of ownership in real-world use.
SMART Communications
As a cloud-hosted CCM platform, SMART Communications is designed to be scalable and is often used by businesses in security-conscious industries. A tool that helps users manage customer conversations across multiple channels, including automated document generation. SMART’s API architecture facilitates direct integration with today’s popular business systems.
OpenText Exstream
Built with the goal of transforming customer communications into personalized experiences, OpenText Exstream is a scalable solution for large enterprise organizations. It offers AI-powered personalization and omnichannel communication features. Custom email, text, and web content can be easily generated with processed data. Most powerful when used with the broader OpenText ecosystem, and also supports connections to third-party applications. However, it is less user-friendly and potentially more expensive than other tools on the market
Adobe Experience Manager
AEM is a comprehensive digital asset and content management (DAM) platform aimed at large enterprises that require technology-driven customer care processes. Adobe helps organizations manage their content libraries, including document management across multiple channels. The main strength of the tool is its seamless integration with the Adobe Creative Cloud and Experience Cloud suites. The downside to this tool is its complexity, which can make it difficult to implement even for experienced users, and its high cost.
How to Get Started with Automated Document Processing
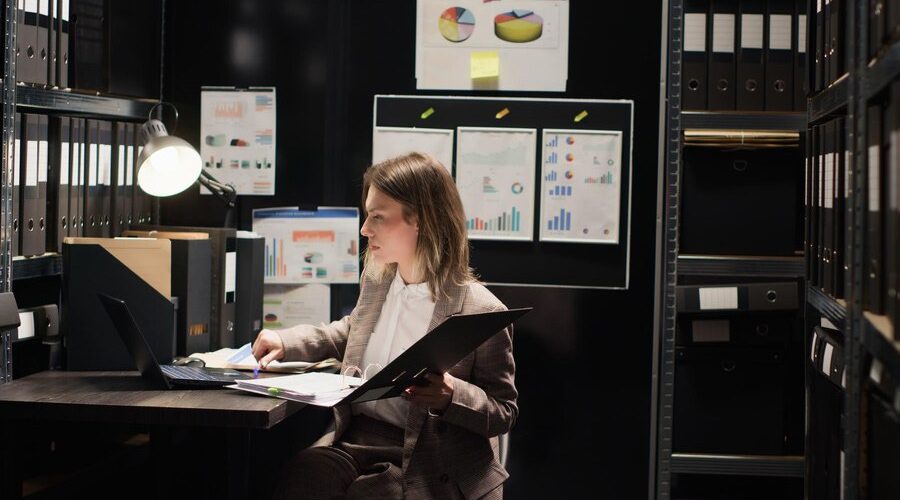
To implement automated document processing, you need to understand the following steps to be able to apply it correctly and effectively
- Assess current needs: Start by reviewing and reviewing your company’s existing document workflows. Identify the key data types and volumes of documents that need to be processed, identify bottlenecks and inefficiencies, and measure current processing times and errors when performing manual tasks. With proper assessment, you can see improvements after implementing automated document processing.
- Define goals: After the assessment, set clear, measurable goals for the document processing automation project. Goals should align with business needs, such as reducing processing time by a specific percentage, reducing error rates, achieving cost savings, etc.
- Choosing the right solution: Next, try to evaluate automated document processing vendors and tools that match your defined requirements and goals. Key criteria are the ability to handle the data types and volumes of documents, the accuracy of the solution (OCR/AI performance), the ability to integrate with existing systems (ERP, CRM via API), scalability, security features and licenses (GDPR, HIPAA), ease of use, deployment options (cloud/on-premises), vendor support, and overall cost-effectiveness (TCO vs ROI).
- Implementation and Integration: Now you will begin the process of automating your chosen document workflow. Vendors will now configure the collection methods, classification rules, extraction models (which may require ML training), validation checks, and human workflows in the loop.
- Train Users: After deployment, vendors will need to provide comprehensive training to employees on how to use the new automated document processing system, handle exceptions, and understand how the system works.
- Monitoring and Optimization: Once deployment is underway, ongoing monitoring of key performance indicators (KPIs) against targets will reflect the effectiveness of the solution. At this point, user feedback will need to be collected and the system configuration periodically reviewed and refined so that the solution can deliver ongoing value to the company or organization.
FAQs
In this section, DIGI-TEXX will clarify frequently asked questions about automated document processing.
What is Intelligent Document Processing? (IDP)
Intelligent Document Processing (IDP) is a form of automated document processing that uses a combination of technologies: Artificial Intelligence (AI), Machine Learning (ML), Natural Language Processing (NLP) and Computer Vision. IDP aims to understand the content and context of documents as well as humans. IDP systems can automatically classify different types of documents, extract contextual data even when the format changes, intelligently authenticate information and learn/improve over time.
=> See more: Innovative Intelligent Document Processing Solutions for Businesses
What is the difference between IDP and NLP?
IDP (Intelligent Document Processing) and NLP (Natural Language Processing) are not interchangeable; Instead, NLP is a key component technology that drives IDP solutions. NLP is a subset of AI that focuses on enabling computers to understand, interpret, and process human language. IDP, on the other hand, is an overall solution or application that automates the document lifecycle (collection, classification, extraction, validation, integration). IDP uses NLP, along with other AI technologies such as ML and Computer Vision, to intelligently process the content in documents, especially unstructured text.
What is an example of document automation?
Document processing automation is the processing of supplier invoices in accounts payable. The automated workflow typically includes:
- Collect: Invoices received via email or scan are automatically imported.
- Classification: The system identifies the document as an invoice.
- Extraction: AI/OCR extracts key data such as supplier name, invoice number, date, line item, and total amount.
- Validation: Data is checked against the supplier database, purchase orders (PO matches) and payment is processed.
- Integration/Workflow: Validated data is automatically entered into the accounting/ERP system. Matched invoices can be routed for automatic approval, while exceptions (mismatches, errors) are flagged for human review (HITL).
Examples include processing mortgage applications, insurance claims, patient admission forms, HR documents and legal contracts.
What is Automated Processing?
Automated processing is a broad term that refers to the use of technology (software, hardware or both) to perform tasks that require little or no human intervention. The main goal is to increase efficiency for the company, speed up operations, and reduce costs. This process focuses on streamlining workflows by automating repetitive activities based on established rules.
Conclusions
In short, automated document processing is a step forward for any business. If you plan to spend wisely, the benefits are undeniable: increased efficiency, superior data accuracy, enhanced security, significant cost reduction, and faster decision making. Are you ready to harness the power of document process automation? DIGI-TEXX offers solutions such as Advanced Automated Document Processing. Contact DIGI-TEXX today to find out how our tailored solutions can accelerate your digital transformation and optimize your company and organization’s document processing automation.
=> Read more: