The data analysis process is extremely important because it helps businesses make informed decisions instead of relying on intuition or random guesses. In this article, DIGI-TEXX will guide you through data processing and analysis and review 6 steps from defining goals to applying, thereby reducing costs and creating competitive advantages in the market.
=> See more: How Data Processing Services Drive Customer Insights and Engagement
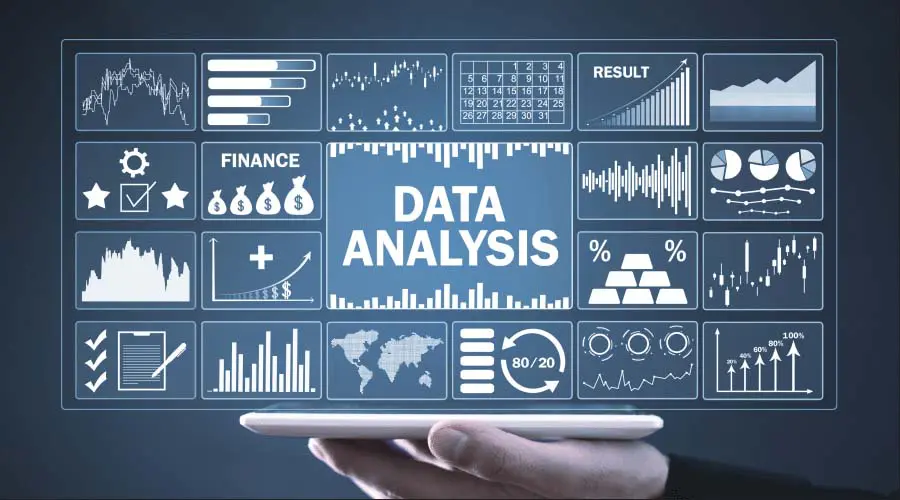
What is the data analysis process?
The data analysis process includes data inspection, cleaning, transformation, and modeling. It provides useful knowledge to support the final decision-making process for an object or event. These steps help convert raw information into knowledge so that action can be taken to solve the problem.
The data analysis process is extremely important because it helps businesses make informed decisions instead of relying on intuition or random guesses.
An easy-to-understand example follows: A company operating on an e-commerce platform is experiencing a decline in sales. In this situation, using the data analysis process is extremely necessary. First, data analytics will help identify customer behavior, evaluate the effectiveness of marketing campaigns, and find the root causes of declining sales. Similarly, healthcare providers will also be able to implement data analytics to improve patient outcomes, perhaps by analyzing the effectiveness of treatments and allocating resources more appropriately.
Why is data analytics necessary today?
As data is the most important factor in today’s time, organizations and large companies generate more than 120 zettabytes of data every day. Without an effective and systematic data analytics process, it will be difficult for businesses to convert this huge amount of data into important information to make decisions for the business. The explosion of big data, cloud computing, and hosting & storage has made data processing and analysis more accessible than ever.
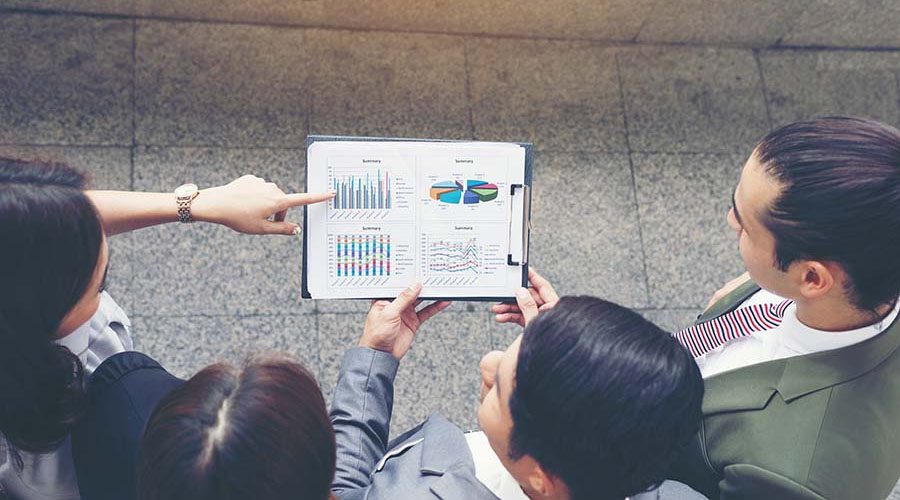
A modern and systematic data analytics process will help:
- Make decisions based on data instead of relying on intuition or outdated practices.
- Identify trends and patterns that can be missed by the naked eye in complex data sets.
- Reduce costs by increasing the efficiency of operations and optimizing resources.
- Create a competitive advantage in a rapidly changing market with timely knowledge and information.
- Innovate the way you do things due to a deeper understanding of customer needs and market concerns.
- Minimize & manage risks by identifying potential issues before they become major, out-of-control issues.
- Easily track performance against initial goals through real-time metrics.
In particular, in the context of accelerating digital transformation, good data analysis skills have become a must-have competency rather than a specialized skill. Organizations that lack a data analysis process framework are certainly at risk of falling behind competitors who leverage data analysis as a key strategy.
=> Real-Time Data Scraping: Insights Instantly
What are the 6 basic steps of the data analysis process?
1. Define the Goal
The first and most important step in any data analytics process is to clearly define the problem you or your organization is trying to solve. This involves formulating specific, measurable questions that will guide the subsequent analysis. Without a clear goal, analytics efforts can waste resources and generate insights and information that are not relevant to the final decision.
When defining the goals for data analysis, consider the following factors:
- Specific business issues that need to be addressed
- Key metrics for a successful project
- Stakeholder needs and expectations
- What decisions will be made based on the results of the data analysis?
- Time and resources for data analysis
To take a simple example, instead of setting a vague goal like ‘improve sales’, a goal could be ‘identify the factors that caused the 15% decline in sales in the last quarter in the southwest region and recommend three strategies that can be improved’.
2. Collect Data
Once you have a specific goal, the next step involves collecting data that will involve a variety of sources. The data analysis method you will use will depend on the collection of the appropriate information. Data collection should be purposeful, focusing on collecting information that will directly support your analysis goals.
Data sources may include:
- Internal databases and customer relationship management (CRM) systems
- Customer surveys and feedback forms
- Website metrics and how users interact with the site
- Social media engagement metrics
- Market research and industry reports
- Transaction history and sales statistics
- IoT devices and sensor data
- Public data and government decrees/policies
The quality of the process will directly depend on the quality of the data collected before the analysis begins. Therefore, companies & organizations must ensure proper data governance, including documenting data sources, collection methods, and compliance with confidentiality.
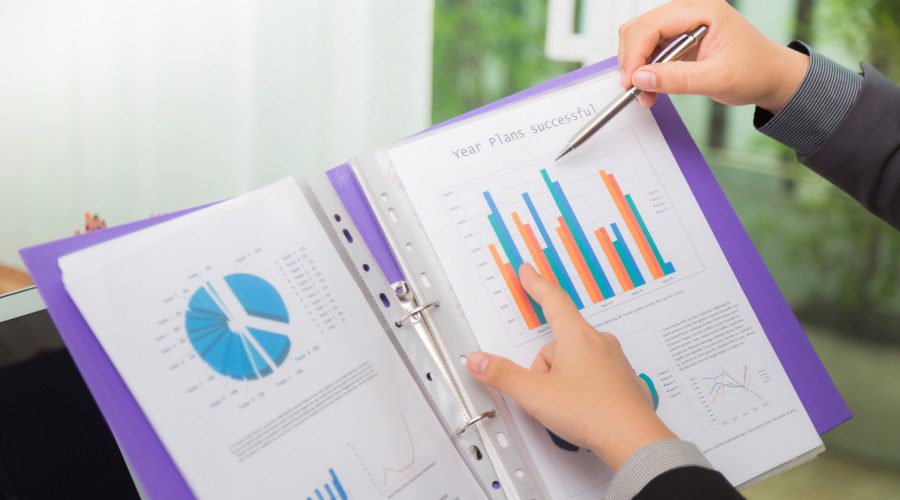
=> You might concern: Automated Document Processing: Revolutionizing Business Efficiency
3. Data Cleaning
Data cleaning is the most important but time-consuming stage of the data analysis process. Raw data often contains errors, duplicates, missing data, and inconsistencies that must be resolved before analysis. Research shows that data scientists and filter scientists spend up to 80% of their time on data preparation activities before starting analysis.
At this point, people will look to automated data processing tools. Automatic data processing can help optimize these steps in the following ways:
- Eliminate duplicate values that can skew results
- Handle missing values in data by assigning values or deleting
- Standardize formats and units across different data sources
- Detect and correct unusual or outlier data points.
- Normalize data for more consistent analysis
- Validate data against given rules: business rules or logical constraints
Effective data cleaning requires both technical skills and domain knowledge in that field. Automatic data processing systems increasingly incorporate machine learning to identify data quality issues and suggest fixes. The time spent on this step will become more optimized over time.
=> See more:
4. Data Analysis
After data preparation, this core step involves applying statistical techniques and methods to analyze data patterns, find relationships between them, and provide necessary information. Modern analysis methods increasingly utilize artificial intelligence data processing (AI data processing) to improve the ability to analyze and process more complex data types:
AI data processing will use machine-learning algorithms and natural language processing to carry out the following steps:
- Identify complex data patterns in large data sets that traditional methods will miss or make mistakes.
- Make predictions based on historical data with higher accuracy
- Automatically classify multidimensional information.
- Process unstructured data such as text, images, and videos, which contain valuable information.
- Detect anomalies in data.
- Generate real-time information due to the ability to access data on the Internet.
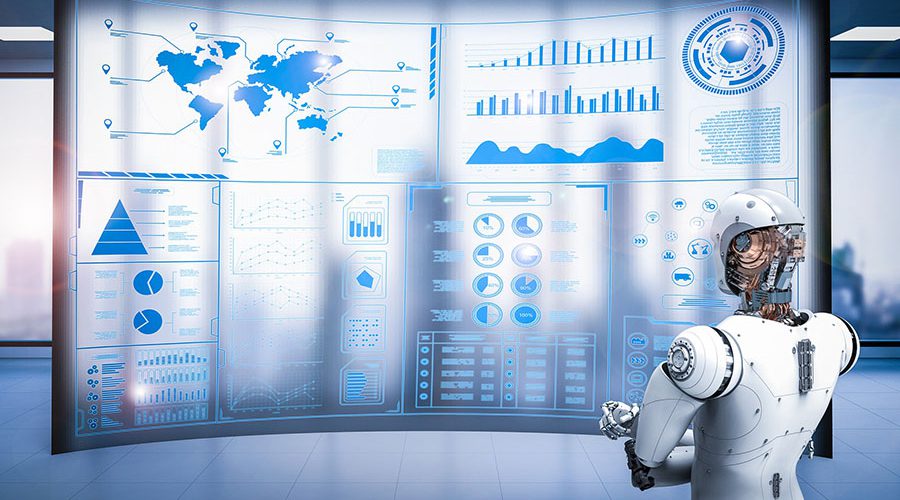
The analysis phase can use many different methods depending on the goal of data analysis. The following methods can be referred to:
- Descriptive statistics of small data sets to summarize the characteristics of large data sets.
- Inferential statistics to test initial hypotheses and conclude.
- Analyze new data to find new information.
- Confirmatory analysis to validate existing theories.
- Time-based analysis to identify new trends over time.
- Network analysis to better understand the relationships between elements in the network.
5. Data Interpretation, Visualization, and Data Storytelling
After analysis, the raw results must be interpreted or transformed into compelling visuals to communicate to decision-makers. Even the most sophisticated data processing and analysis will be worthless if decision-makers cannot understand the implications of the process that has been analyzed.
Steps include:
- Create data charts, providing relevant information to decision-makers.
- Create interactive tables and charts that easily reveal new information.
- Storytelling with data and explaining the important things behind the numbers
- Link the newly analyzed findings to business goals and KPIs.
- Highlight new information that can be immediately acted upon, thereby driving decision-making.
- Create a context, a hypothetical environment that is appropriate for interpreting the results.
However, the analysis will not be 100% accurate, and it is necessary to provide limitations and confidence levels in the data you have analyzed.
Effective data-based storytelling will transform abstract numbers into a compelling story. For example, instead of simply reporting that the customer churn rate is 23%, the data-based story could explain how price changes affect customer segments. Therefore, what customer retention strategies are needed. At this point, you can rely on AI data processing models to further reference the results.
6. Embrace Possible Failure
Finally, we must all acknowledge that data analysis is an iterative process, and there will be failures that you will recognize when looking at the data. By noting challenges, tried and failed methods, and remaining limitations, analysts can refine their methods for current results, as well as for future analyses.
This step will include actions such as:
- Reviewing the performance of the analysis based on the initial goals set
- Noting limitations and assumptions that affect the results
- Taking immediate action that could change the results
- Collecting metrics on changes made to measure their impact
- Optimizing and continuously improving the data analysis process
- Creating a common knowledge repository to store lessons learned
- Creating a process to develop, test, and repeat similar analyses
Companies and organizations with a robust data analysis framework will recognize that their initial hypotheses will often be incorrect, but these ‘failures’ will generate more valuable insights in the future. Creating an acceptance mentality for further analytical experimentation will motivate more innovative approaches to solving data problems in the future.
Types of Data Analysis Techniques
Many different types of analysis serve different purposes depending on the end goal of the data analysis process, including:
Diagnostic Analysis
This type of analysis answers the question: Why did something happen? This will be solved by looking at causal relationships and root causes in data over time. Diagnostic analysis goes deeper into the descriptive, seeking to understand the underlying factors that drive the visual outcome. For example, this type of analysis would help you understand why customer churn increases after a price change by analyzing customer segments, the services they use, and the data collected as customers use the service.
Predictive Analysis
This type of analysis uses statistical models and machine learning to predict future outcomes based on data over time. This analysis will form the core points to be included in the AI data processing system, helping companies and organizations predict changes and prepare appropriate adaptive solutions. Examples of the results of this analysis include revenue forecasting, media risk assessment, and customer demand prediction to provide appropriate service maintenance schedules without affecting the usage process.
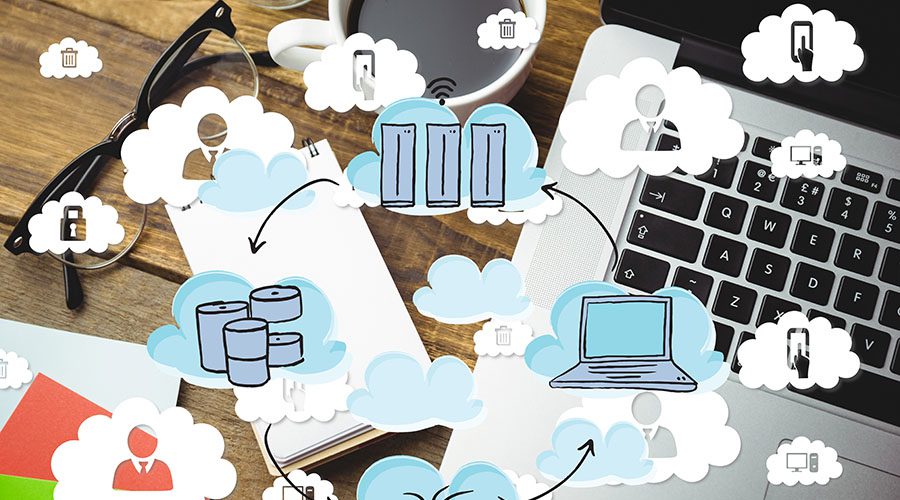
Prescriptive analysis
This analysis will go beyond predictions to propose the most optimal actions. This technique will produce results that are recommendations for the best course of action based on different scenarios, constrained by many factors. Prescriptive analysis will use optimization algorithms, simulations, and AI data processing to evaluate possible decisions and propose the most optimal strategy. An example of this outcome is providing the ideal cross-selling product package to optimize profits while minimizing inventory costs.
Inferential Analysis
This type of analysis is based on sample data collected from surveys or information collected on a certain sample. This type of analysis helps organizations generalize the majority of information when checking all the existing data is not possible. Inferential analysis will test the hypothesis, and then determine the confidence interval and regression analysis. For example, for this type of analysis, survey response data from 500 customers to make reliable predictions about the preferences of a customer file of 50,000 people.
Tools for Data Processing and Analysis
To be able to process and analyze data in a modern way, it is necessary to rely on tools; powerful tools today are capable of handling different aspects of the analysis process. Below are some popular tools that you can refer to.
Python: This popular programming language with libraries such as Pandas, NumPy, and sci-kit-learn supports optimal statistical analysis and machine learning. The flexibility and popularity of Python make it an ideal tool for complex data processing and analysis tasks, from data cleaning to deep learning.
R: Meanwhile, R specializes in statistical computing and data visualization & graphics, with customization for many different analysis techniques. This is a popular tool among scientists who often use statistics in the data analysis process.
SQL: Suitable for research techniques that require impact on databases and database relationships. To date, SQL is still the foundation for structured data retrieval and forms the basis for many different data processing and analysis processes.
Power BI: As one of the applications in the Microsoft 365 ecosystem, Power BI helps visualize interactions between data and predict business trends. Power BI allows non-technical users to participate in the data analysis process through an intuitive and easy-to-understand interface.
Tableau: Tableau is an application that helps visualize data, convert data into information, and take action through an intuitive drag-and-drop interface. The strength of Tableau is the creation of data visualization, which is of great value to the data interpretation and data storytelling process of the data analysis process.
Excel: Although there are many visual data analysis tools available today, Excel is still a versatile choice for quick analysis and creating data charts that are familiar to the majority of users.
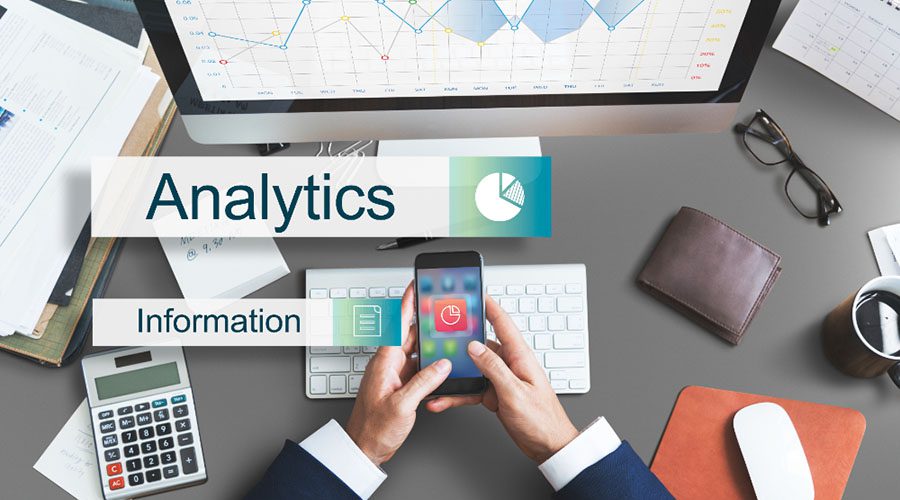
RapidMiner: This is a platform specializing in raw data preparation, machine learning, and model deployment with intuitive workflows. RapidMiner will help simplify complex AI data processing techniques into simple ones for business analysts to make easier decisions.
Apache Spark: Specialized in large-scale data analysis. Apache Spark is widely used by professionals who regularly process huge data sets that exceed the capabilities of traditional tools, allowing for big data analysis.
It can be seen that data analysis tools will continue to evolve; with the increasing integration of AI data processing capabilities, AI will automate routine tasks and enhance analytical capabilities. Companies and organizations will have to use many tools in the data analysis process, choosing the most suitable solution for each step of their analysis.
Conclusion
This article goes through the data analysis process, pointing out 6 important steps from defining goals to accepting failure. This article provides a comprehensive roadmap to help businesses improve their ability to make data-driven decisions, optimize operations, and create competitive advantages. Contact DIGI-TEXX today for advice on personalized data processing and analysis solutions, helping your business maximize the potential of existing data warehouses and achieve achievements through digital transformation applications.
=> You might like:
- Survey Data Processing Services: Transforming Raw Data into Insights
- The Advantages of Outsourcing Your Data Processing Needs
- Data Entry Service Provider | Elevating Your Business Efficiency
- Data Extraction Automation: Streamlining Your Data Workflow
- Document Processing Companies: Key Benefits for Modern Enterprises