As organizations amass increasing volumes of data, data quality management grows ever more critical. Data serves as the backbone of your organization, and effective data quality management integrates culture, technology, and data to produce reliable, actionable outcomes. So, what is quality data management, and what should businesses do after integrating their data? Let’s explore this in the following article with DIGI-TEXX.
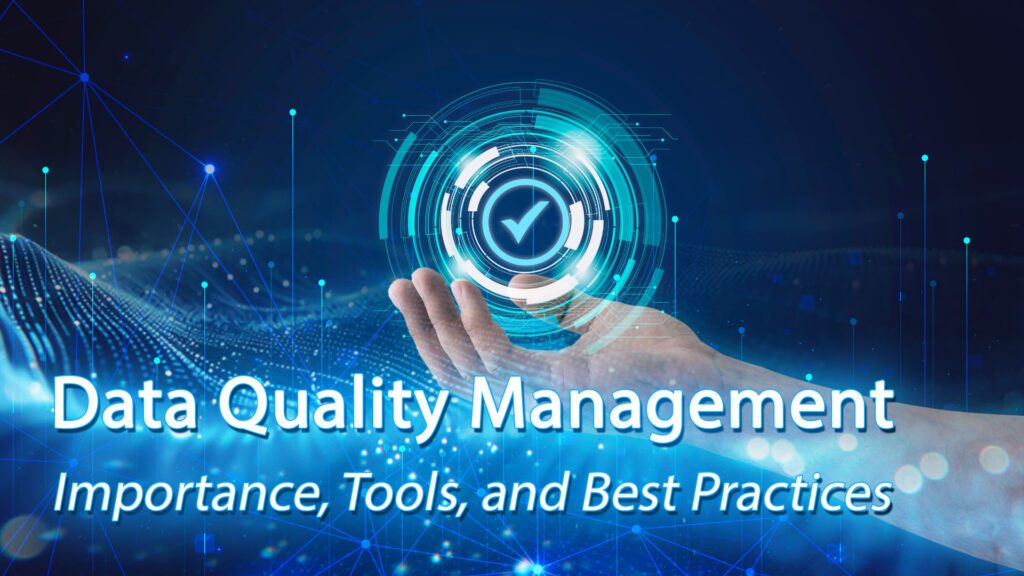
What is Data Quality Management?
Data quality management is a framework of practices designed to ensure the maintenance of high-quality information. It encompasses data acquisition, the application of advanced data processes, and the efficient distribution of data. Additionally, it involves managerial oversight to effectively manage the data in your organization.
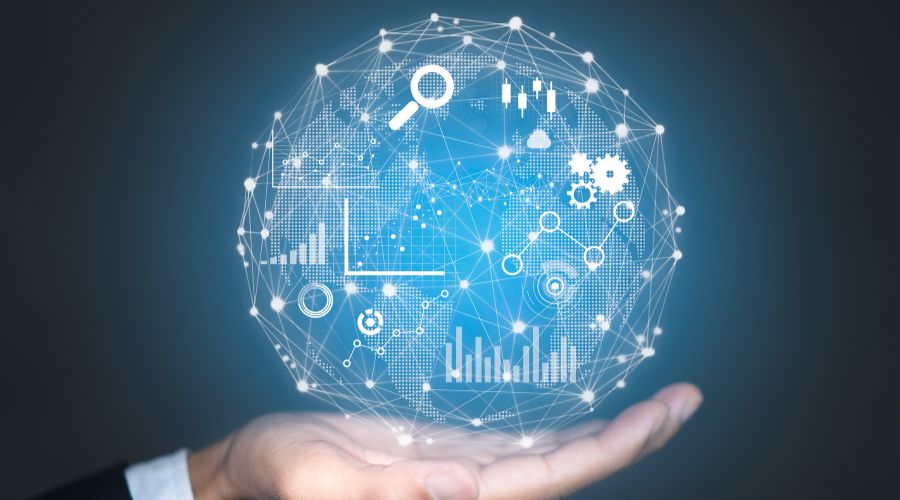
Benefits of Data Quality Management
Data management and data quality a critical processes for effectively understanding and utilizing your data, which can ultimately improve your business outcomes. Here are 3 benefits of data quality management that DIGI-TEXX wants to provide to you:
1. Data Quality Framework for Business Initiatives
Robust data quality management establishes a reliable backbone for all business activities. Inaccurate or obsolete data can result in mistakes and flawed decision-making. A data quality management program creates a structured framework across departments, establishing and enforcing rules that ensure data quality.
2. Accurate Information and Cost Reduction
Accurate and up-to-date data offers a clear view of your company’s daily operations, ensuring confidence in both upstream and downstream applications that rely on this data. Effective data quality management also reduces unnecessary costs. Poor data quality can lead to expensive mistakes and oversights, such as losing track of orders or overspending. Data quality management creates a solid information foundation, enabling better insight into organizational performance and spending by having a clear understanding of your data.
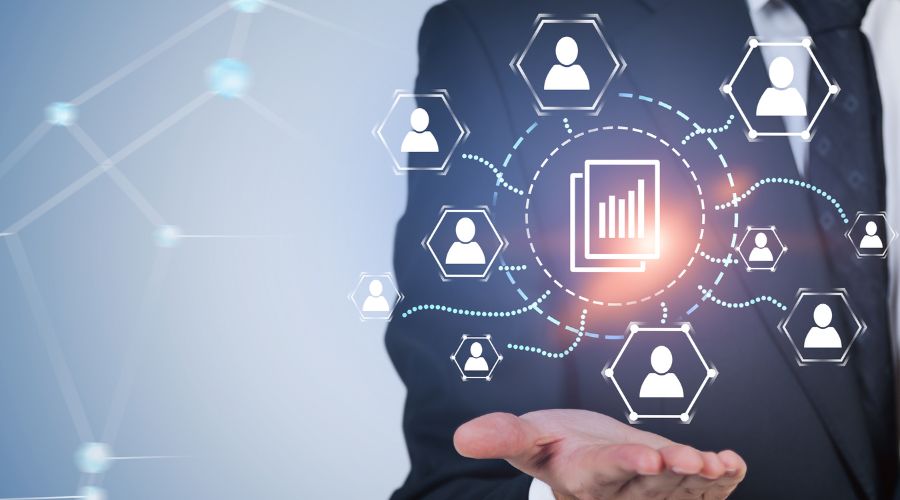
3. Compliance and Risk Management
Strong data quality management is essential for ensuring regulatory compliance and meeting risk management goals. Effective data governance requires well-defined procedures, clear communication, and high-quality data. For instance, a data governance committee may set guidelines on what constitutes “acceptable” data health. But how do you define this in the database? How do you monitor and enforce these policies? Data quality management translates these policies into action at the database level.
Data Quality Management Process
Data Quality Management processes will vary depending on how data is utilized across different business areas. Therefore, your framework should have a comprehensive vision while also providing tactical guidelines that allow each team or activity to aim for the level of quality required to ensure safety, efficiency, compliance, cost-effectiveness and competitiveness. This same approach applies when building your Master Data Management framework, which should emphasize the fundamental rules for data acquisition, usage, storage and access.
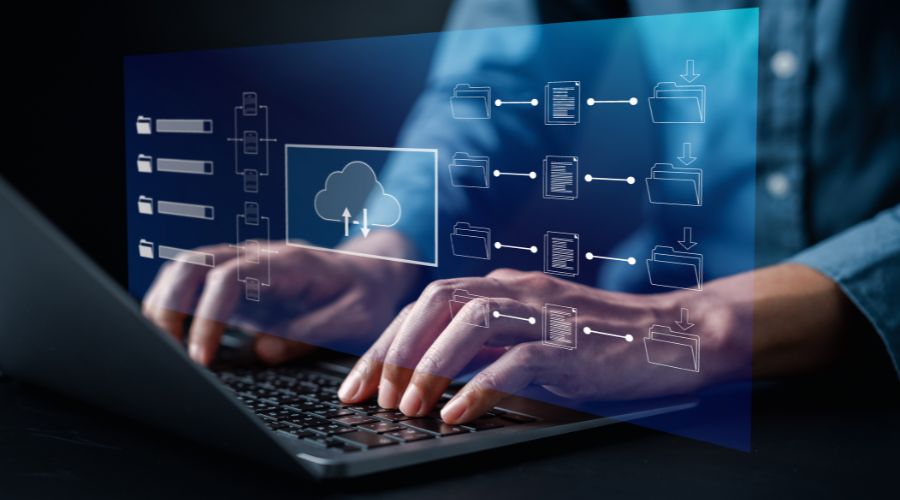
Data Management processes vary depending on how data is utilized
Key Features of Data Quality Management
A robust data quality program utilizes a system with various features that enhance the reliability and trustworthiness of your data. Below are 3 key features of data quality and management:
Data Cleansing and Correction
Removing outdated data enhances an organization’s profile by eliminating unnecessary information, as this process helps manage problems such as duplicate records, undefined data types, and nonstandard data formats. It enforces data sanitation policies required to attain insightful knowledge from the data sets. More importantly, data cleansing also aids in the construction of data hierarchies alongside definitions and reference data tailor-made to suit your specifications.
=> Check out our data cleansing services.
Data Profiling and Discovery
Cleansing and monitoring data includes the application of profiling, which in turn aids in the verification of data against the standard statistical measures, relationships are exposed, and comparisons against descriptions of data are made. The activities in data profiling assist in the identification of patterns, the systematization of data, inconsistencies, and provide better insights into data.
=> Essential B2B Data Cleansing Services to Improve Your Database
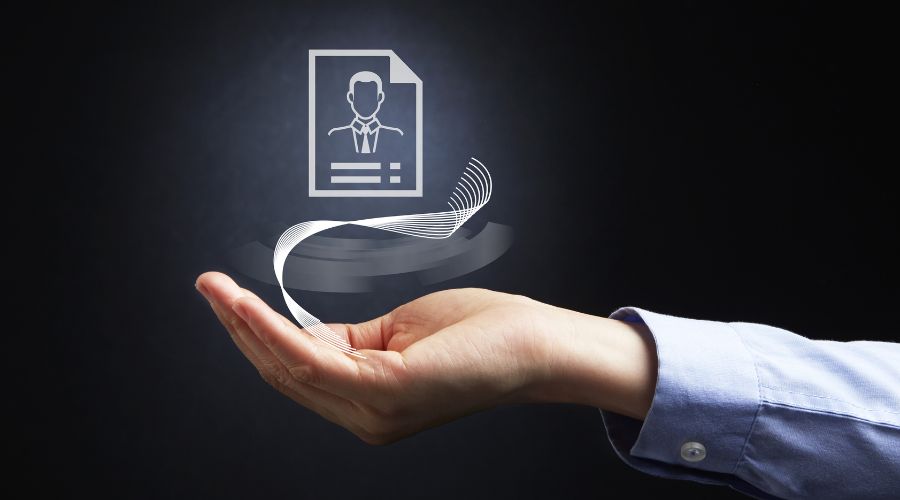
Cleansing and monitoring data include the application of profiling
Data Validation and Standardization
Establishing business validation rules with a corresponding business glossary, lineage is a preventive measure for managing poor-quality data that can negatively affect the organization. It embraces the creation of descriptions, synonym sets, and phrase requirements, generalizing terms across versatile systems. Validation can be performed against standard statistical benchmarks designed for universal criteria or customized rules designed for the matter at hand to ascertain the quality of data.
=> Essential Address Validation Software for Accurate Data Entry
Data Monitoring and Alerting
Continuously tracking data quality metrics and KPIs, Data Monitoring and Alerting proactively detects anomalies, errors, or deviations from predefined standards, notifying stakeholders through automated alerts. It leverages real-time or scheduled checks, customizable rules, and AI-driven anomaly detection to ensure data accuracy, completeness, and timeliness.
Data Governance and Collaboration
Data Governance and Collaboration establishes a framework of policies, roles, and processes to ensure data is managed consistently, securely, and in alignment with organizational goals. It involves defining clear ownership, access controls, and accountability for data assets while fostering cross-functional collaboration through shared glossaries, metadata, and workflows.
Build a Data Quality Strategy
Improving data quality and developing a data quality strategy requires a balanced approach that integrates people, processes, and technology, along with strong involvement from top-level management.
Data Quality Dimensions
The goal of enhancing data quality is to assess and improve various dimensions of data quality.
- Uniqueness: Prevent duplicates and use detection tools during onboarding and bulk deduplication.
- Completeness: Set clear standards for data collection and audit data regularly, using automated systems for incomplete entries.
- Consistency: Standardize address formats and use location intelligence tools to maintain uniformity.
- Precision: Define necessary precision levels and use geocoding services to improve location data accuracy.
- Conformity: Standardize product data units and provide clear guidelines for data entry consistency.
- Timeliness: Ensure real-time data synchronization and implement regular data refresh protocols.
Accuracy, Validity, and Integrity: Verify data against external sources, use validation rules, and maintain relational integrity with database management systems.
Data Quality Management
Data quality management seeks to implement a well-rounded set of tools and processes to proactively prevent data quality problems and correct or eliminate data that does not meet established Key Performance Indicators (KPIs), thereby supporting the attainment of business goals.
Data quality KPIs are typically evaluated based on core business data assets, considering dimensions such as data uniqueness, completeness, consistency, conformity, precision, relevance, timeliness, accuracy, validity and integrity.
These data quality KPIs should align with the KPIs used to measure overall business performance.
The approaches to prevent data quality issues and perform eventual data cleansing include the following practices:
- Data Governance
- Data Profiling
- Data Matching
- Data Quality Reporting
- Master Data Management (MDM)
- Customer Data Integration (CDI)
- Product Information Management (PIM)
- Digital Asset Management (DAM)
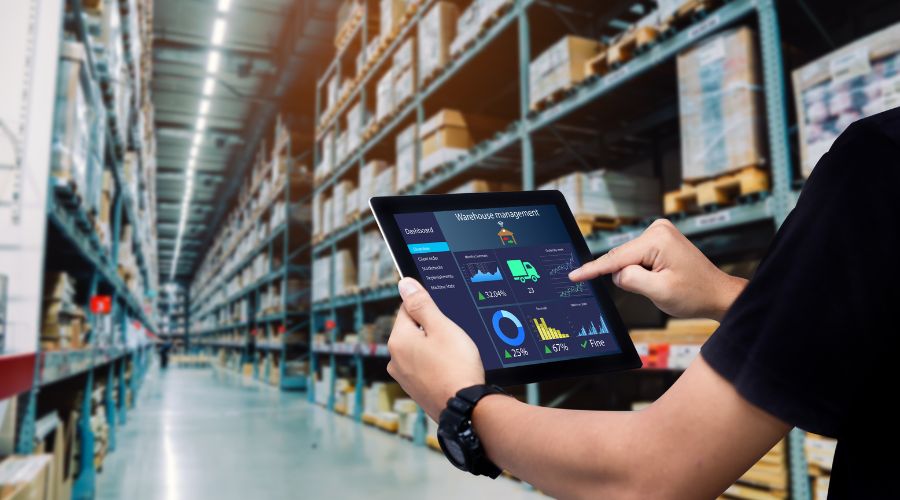
Data quality KPIs are typically evaluated based on core business data assets.
Data Governance
A data governance framework defines the data policies and standards that establish the required data quality KPIs and specify the data elements to be addressed, including business rules supported by data quality measures.
It must also outline the organizational structure needed to maintain data quality, such as a data governance committee, and roles like data owners, stewards, and custodians, tailored to the organization’s needs.
Additionally, a business glossary is an important product of data governance, helping define metadata and ensuring consistent data definitions within the organization and its business ecosystem.
Data Profiling
If you are a data quality manager, it is crucial that those responsible for data quality, as well as those tasked with preventing data issues and cleansing data, have a thorough understanding of the information involved.
Data profiling, often supported by specialized tools, is a method used to analyze data assets in data quality management. These assets are typically created by different individuals following varying business rules and collected for specific business purposes.
In data profiling, the frequency and distribution of data values are analyzed at relevant structural levels. It can also help identify keys linking data entities across multiple databases, and measure the extent to which this is already addressed within individual databases.
=> Understanding the Data Analysis Process: A Step-by-Step Guide
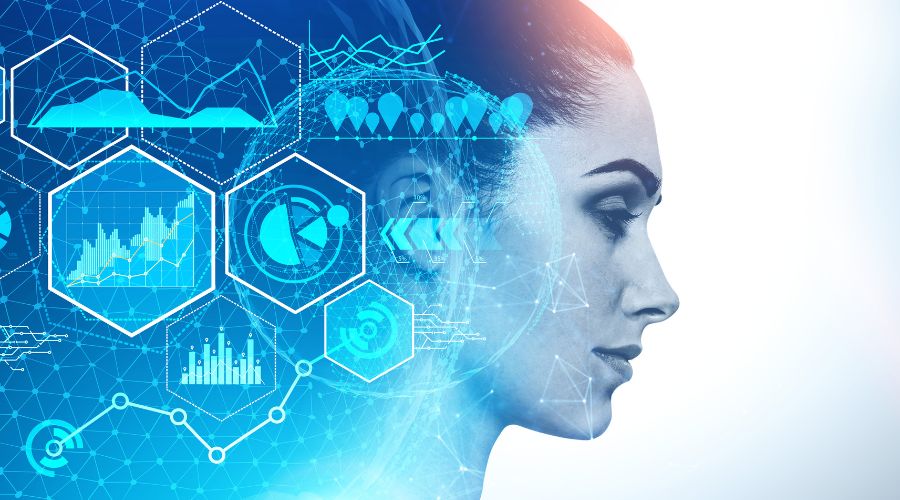
Data profiling is often supported by specialized tools
Data Matching
When processing data quality and management, using exact keys in databases is often insufficient. A common example is the variation in spelling a person’s name due to typos, misunderstandings, or the use of nicknames. The issue is even more complex with company names, which may include mnemonics or legal forms. Similarly, postal addresses can be written in many different ways.
Data matching is a technology that uses methods like match codes, fuzzy logic and increasingly machine learning, to determine whether two or more data records refer to the same real-world entity. This method can be used for deduplicating a single database or identifying matching entities across multiple data sources.
Data matching frequently involves data parsing, which involves separating names, addresses, and other elements into individual, distinct components.. For example, a full address might be split into building name, unit, house number, street, postal code, city, state/province, and country, and further standardized by using consistent abbreviations.
=> Essential Data Parsing Software to Improve Data Processing
Data Quality Management Reporting and Monitoring
The results from data profiling can be used to measure quality data management KPIs based on the relevant quality dimensions for an organization. Data matching results are highly useful for evaluating the uniqueness of data. Furthermore, keeping a data quality issue log is beneficial for recording identified data quality issues and monitoring the progress of preventive measures and cleansing efforts. Organizations prioritizing data quality often use a data quality management dashboard to display KPIs, monitor trends in their measurements, and track issues logged in the data quality issue log.
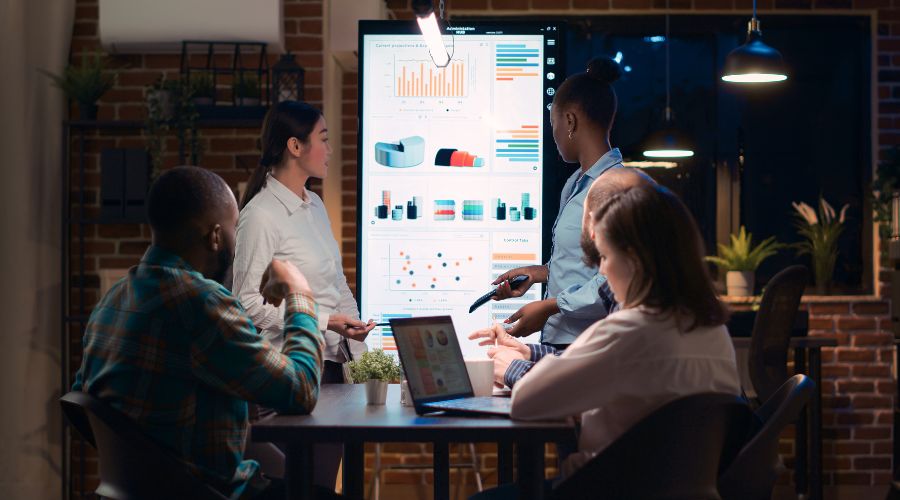
Data profiling can be used to measure data quality
Master Data Management (MDM)
To prevent recurring data quality issues and avoid constant data cleansing efforts, most organizations will need to implement a master data management (MDM) framework.
MDM and Data Quality Management (DQM) are closely linked disciplines, both integral to the same data governance framework and sharing roles like data owners, data stewards, and data custodians. Data profiling typically focuses on master data assets. When performing data matching, the results should be retained within master data assets to manage merged and purged records, as well as the survivorship of data attributes related to those records.
Customer Data Integration (CDI)
Customer master data in many organizations is sourced from various applications, such as self-service registration sites, CRM systems, ERP applications and customer service platforms.
In addition to setting up the technical infrastructure to consolidate customer master data into a single source of truth, significant effort is required to maintain the quality data management of that source. This includes data matching and implementing sustainable processes to ensure data completeness, consistency, and accuracy.
=> Comprehensive Guide to Choosing CRM Data Cleaning Solutions
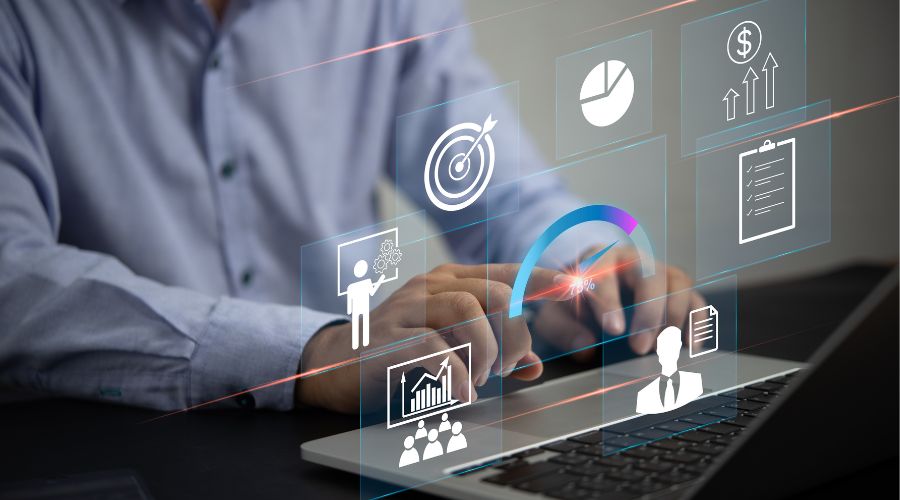
CDI in many organizations is sourced from various applications
Product Information Management (PIM)
As a product manufacturer, align your internal data quality KPIs with those of your distributors and merchants to ensure your products are selected by end customers at every supply chain touchpoint. This involves ensuring data completeness and other quality dimensions in the product data syndication process.
As a merchant, you collect product information from various suppliers, each with their data quality KPIs (or none at all). Merchants must collaborate with suppliers to ensure a consistent and high-quality product data flow that meets the merchant’s data quality KPIs.
Digital Asset Management (DAM)
Digital assets, such as images, text documents, videos and other files, are often used alongside product data. From a data quality standpoint, key challenges involve accurate metadata tagging and maintaining the integrity of data assets. For example, a product image should clearly depict the product and provide sufficient context, rather than just showing the product without clarity or detail.
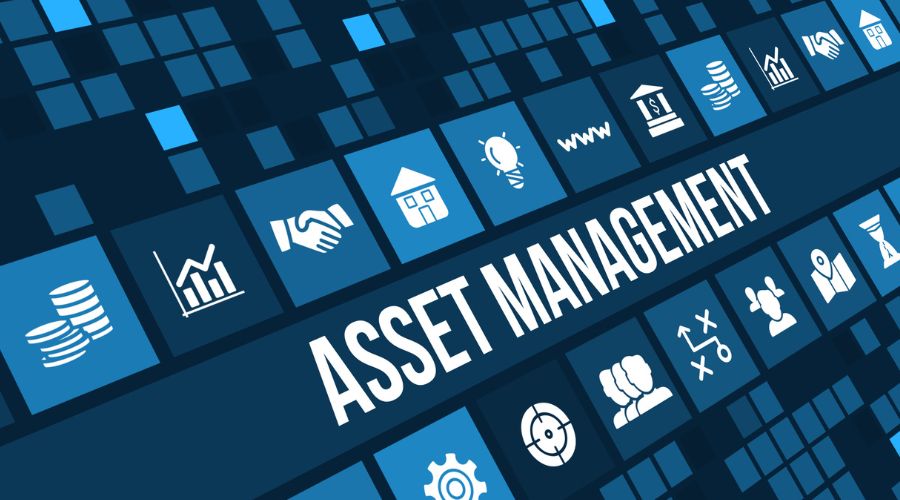
DAM are often used alongside product data
The Impact of AI and Automation on Data Quality Management
Data quality management (DQM) is vital for informed decision-making, but the volume and complexity of AI-era data challenge traditional methods due to:
- Hidden inconsistencies and inaccuracies affecting analytics and decisions
- Slow manual remediation is hindering business agility
- Scalability issues with manual, rule-based approaches are causing bottlenecks and quality degradation
Organizations now use AI-driven automation to enhance DQM by:
- Automatically detecting anomalies and quality issues with AI tools
- Using machine learning to quickly identify and fix errors, inconsistencies, and duplicates
- Implementing real-time monitoring systems to alert stakeholders of quality deviations
=> See more: Essential Automated Data Processing Services to Enhance Efficiency
Data Quality Management Best Practices
If you wonder how to manage data quality, organizations should adopt these best practices:
- Secure enterprise-wide buy-in from employees and departments.
- Define clear data quality metrics.
- Implement data governance to ensure high-quality data management.
- Create a process for employees to report data entry or access issues.
- Develop a step-by-step process to investigate reported issues.
- Initiate regular data audits.
- Invest in comprehensive employee training programs.
- Maintain and update robust data security standards.
- Appoint data stewards at every organizational level.
- Explore cloud-based data automation opportunities.
- Automate and integrate data streams where feasible.
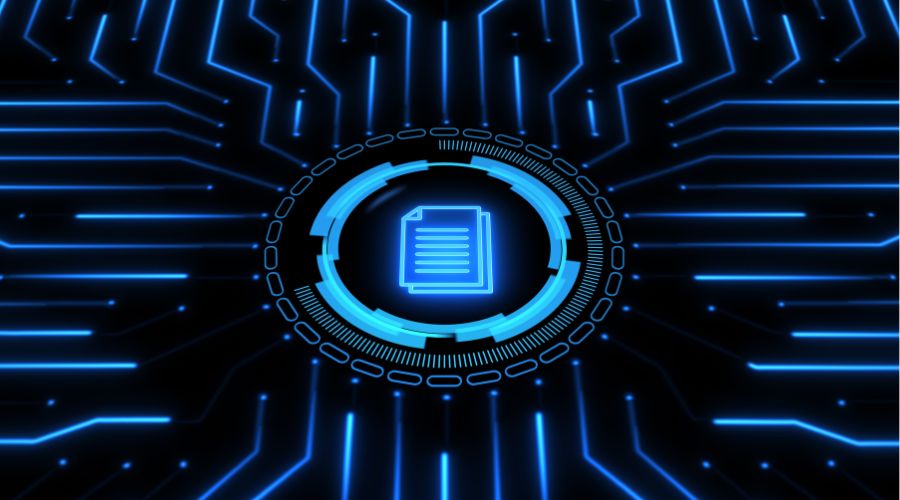
Best Practices for Data Quality Management
Data Quality Tools and Platforms
Here are some data quality management services with their key features and benefits, also with reference links for further reading.
Data Profiling Tools
Such tools assess the health and profile data set by its structure, content, relationships, and by metrics that test interlinking datasets’ as well as data patterns’ interrelationship with each other, including outlier and trend identification. This helps businesses identify gaps that need to be filled to make better, more reliable decisions.
Data Cleansing Tools
Data cleansing tools retrieve logical data sets packed as a collection of records and rules, arrange them and try fetching ordered pieces by matching pedantic, partial ordering and sorting algorithms to arrange the records in a captcha-free systematic, hierarchical manner. Std_03- survey tools accurate and truthful ones assist in improving precision and completeness.
=> Top Data Cleansing Tools for Accurate Data Management
Top Survey Data Processing Services for Accurate Insights
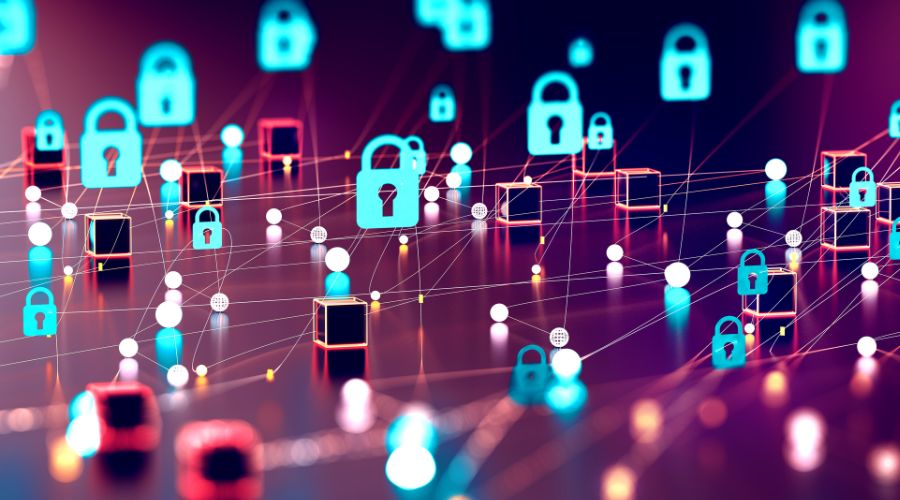
Data Cleansing Tools are set as a collection of records and rules,
Data Matching and Deduplication Tools
These tools are created with special capabilities for identifying approximate matches and redundant data. By employing specific algorithms and designs, these tools promote accuracy and quality of work. This improvesthe data quality management process.
Data Validation Tools
Information as valuable as money – invalidated information can impact an organization financially, therefore, businesses must control data flowing through systems as concepts change each second.
The validation process ensures that specific rules are followed, confirming each data type, range, and format against business rules or validation criteria.
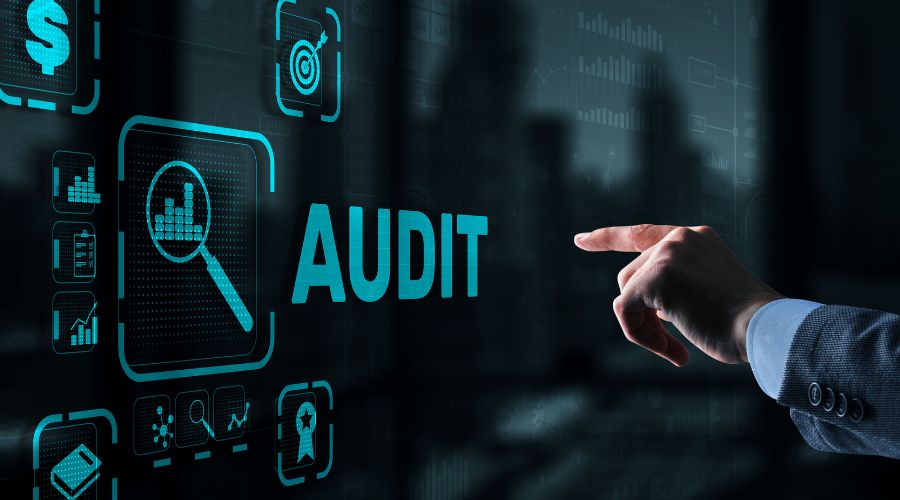
Data Validation Tools process ensures that specific rules are followed
Data Enrichment Tools
These tools augment existing datasets by pulling in extra information from external resources like public records or third-party providers. Enrichment aids in factually augmenting datasets to make them more complete, which in turn makes them more valuable for analytics and decision-making processes.
Data Governance and Data Quality Management Tools
This set of tools creates strategic guidelines for data governance, which include policies, rules, quality frameworks, and stewardship procedures. They define accountabilities and responsibilities to ensure proactive corporate data quality management and compliance with relevant regulations.
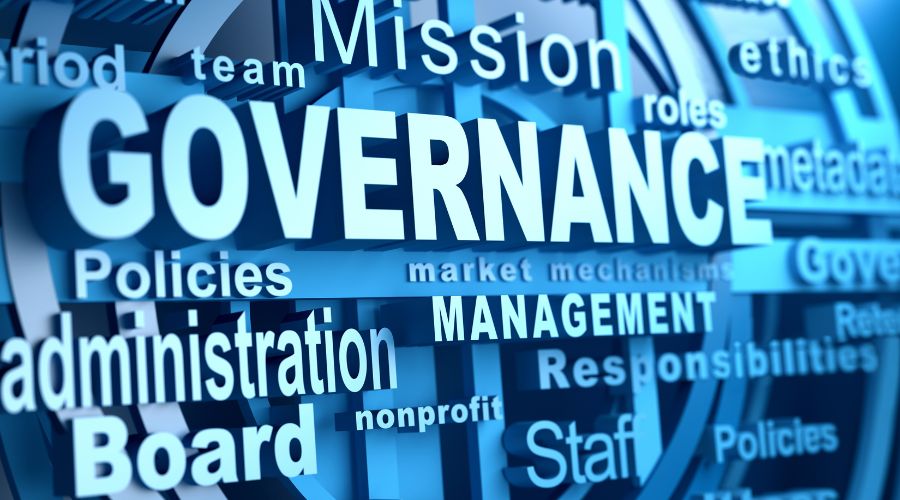
Data Governance and Data Quality Management Tools create strategic guidelines for data.
Data Monitoring and Profiling Tools
Data monitoring tools continuously observe data as it flows into and out of an organization. They detect quality issues in real time, sending alerts to enable immediate action and maintain consistent data quality.
=> How Real-Time Data Scraping Enhances Competitive Analysis
Master Data Management (MDM) Tools
MDM tools create and maintain a unified, reliable source of master data across an organization. They ensure consistency and accuracy for critical data, such as customer, product, or employee information.
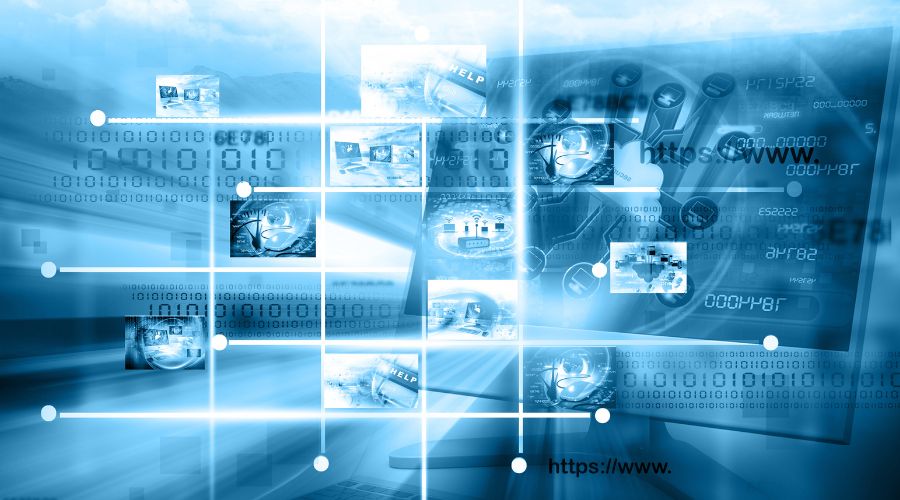
MDM tools create a unified, reliable source of master data
Data Quality Reporting and Dashboards
These tools provide visualization and reporting features to track and share data quality status. They deliver insights into metrics, trends, and areas needing improvement, supporting informed decision-making.
Data Quality Assessment and Profiling Tools
These tools periodically or selectively evaluate data quality, helping organizations identify issues, assess dataset health, and prioritize efforts to enhance data quality and management.
Conclusion
Your data is a critical asset, second only to your people. Without high-quality data, efforts to improve data quality engagement, make informed decisions, support customers, stay competitive, and comply with regulations become challenging or even unfeasible. To ensure superior data quality and streamline your data management processes, explore the innovative solutions offered by DIGI-TEXX.
=> Read more: