Analyzing data to make business decisions is one of the needs that many businesses are interested in, but maintaining high-quality data can be a challenge. This is where a data quality framework comes into play, a well-defined data quality framework not only ensures the accuracy and reliability of data but also helps an organization or company fully exploit the value of data. DIGI-TEXX will delve into the practical aspects of a data quality management framework in this article to clarify the essential tools and real-life use cases to help businesses implement or enhance their data quality management initiatives.
=> See more: Data Quality Framework: Ensure Accurate & Reliable Data
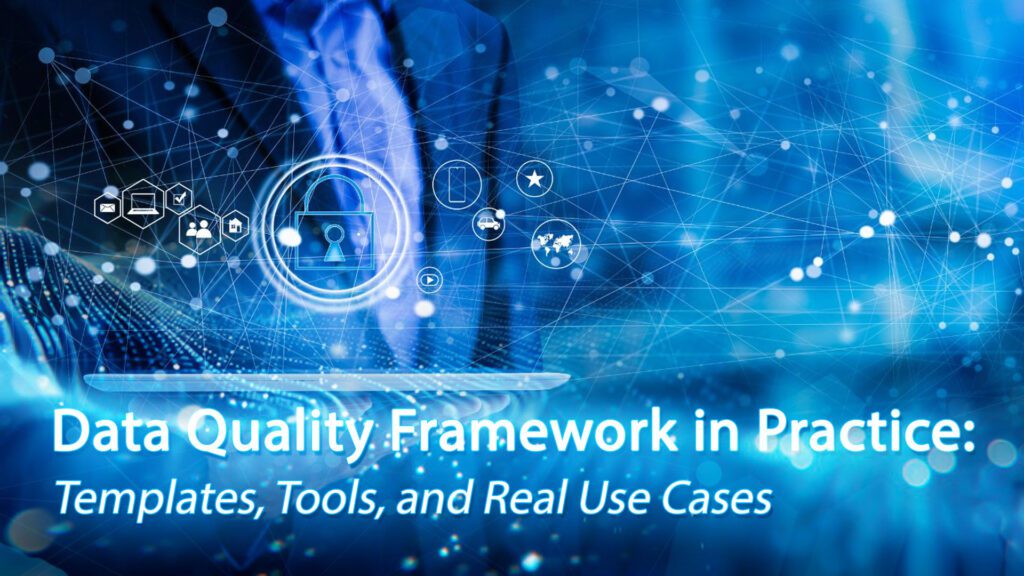
What Is A Data Quality Framework?
A data quality framework is a set of guidelines, processes, standards, roles, and responsibilities designed to manage and improve the quality of data within the company. It can be understood as a blueprint to ensure that all employees in the company are exploiting data that is fit for purpose and supports the company’s business objectives. A data quality management framework is a comprehensive, systematic approach to managing quality throughout the data lifecycle.
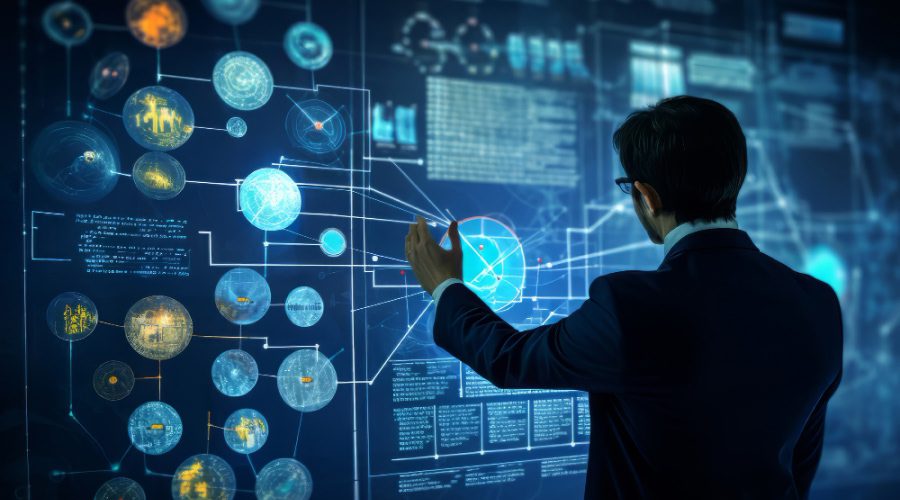
The main goals of using a data quality framework are to measure, monitor, report, and improve data quality. An effective framework will include these key components: accuracy, completeness, consistency, timeliness, validity, and uniqueness, as well as establishing data governance policies and rules, defining data steward roles and responsibilities, implementing data quality tools and technologies, and monitoring processes and dashboards for ongoing reporting.
Essential Elements Of Data Quality Frameworks
A comprehensive framework should be built on criteria that work together to ensure data integrity and reliability. To implement an effective data quality management framework, businesses must pay careful attention to each of these components.
Data Quality Dimensions
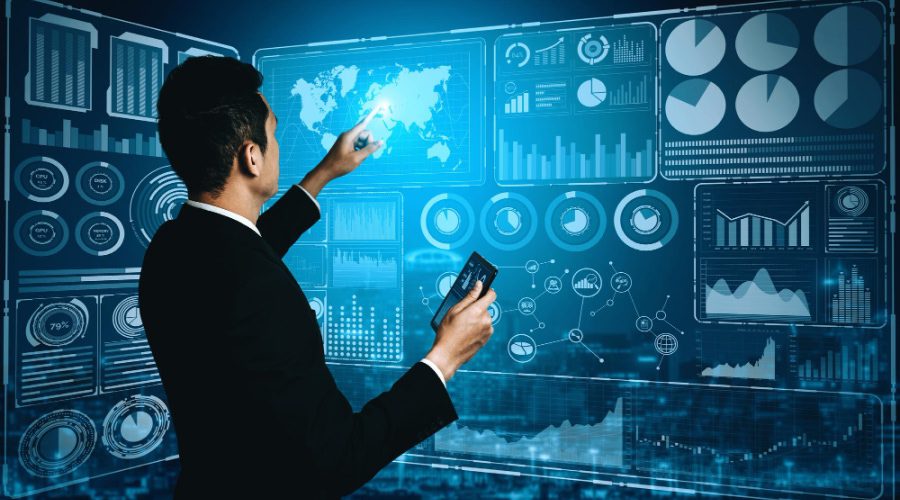
Data quality dimensions are characteristics used to measure and evaluate the quality of data. This criterion is used to assess whether the data is suitable for the original purpose. Focusing on analyzing and calculating these dimensions is the foundation of any data quality framework. This criterion will require clarification of the following points:
- Accuracy: Measures the accuracy of the data. The calculation for this criterion will be by comparing the data with a reliable source or through manual verification. The formula for this content can be understood as follows: (Number of accurate records / Total number of records) * 100%.
- Completeness: This criterion will evaluate whether all the data is necessary to be included in the analysis. Calculation: (Number of required records / Total number of records) * 100%.
- Uniqueness: This criterion ensures that no record is represented more than once in the data set, unless permitted. Calculation: (Total number of records – Number of duplicate records) / Total number of records * 100%.
- Validity: Used to determine whether the data conforms to predefined formats or value ranges. Calculation: (Number of records with valid values for a particular field / Total number of records) * 100%.
- Integrity: Represents the consistency and relationships between different data fields within and between systems, helping to ensure that relationships between data tables are valid. Calculation: (Number of records with valid relationships / Total number of records with relationships) * 100%.
- Timeliness: Measures the extent to which data is available when required and whether it is current enough for its intended use. Calculation: (Number of records updated within the desired time frame / Total number of records) * 100%.
=> Data Accuracy Improvement Solutions | Boost Data Reliability
Data Governance
Data governance establishes the overall framework for managing an organization’s data. This criterion plays an important role in the data quality framework by defining the rules, responsibilities, and processes to ensure data quality. It includes the following points to note:
- Data Ownership & Stewardship: This criterion clearly defines who is responsible for specific data sets and their quality. Data Owners are typically senior business leaders, while Data Stewards are responsible for the day-to-day management of data and maintaining data quality in their respective areas. This ensures accountability and helps address data quality issues effectively.
- Data Policies and Standards: For a data framework to be effective, it is necessary to establish clear, consistent rules, definitions, and standards for creating, collecting, storing, using, and processing it. These rules include data definitions, formats, data quality rules, and security procedures.
Data Quality Management Processes
This refers to the actual processes that are implemented to measure, improve, and maintain data quality. These processes are the core of a data quality framework.
- Data profiling: Analyzing data to understand the structure, content, quality, and relationships between data files. Profiling helps identify anomalies, inconsistencies, and potential threats to data quality that need to be addressed.
- Data validation: The process of ensuring that data complies with defined data quality rules and standards. This typically involves checking data against established definitions, formats, scopes, and constraints that were established in the previous criteria.
- Cleansing: This step reflects data cleansing, fixing, or removing data that is inaccurate, incomplete, irrelevant, or mis formatted.
- Integration: This is the integration of data from different sources to obtain an overall view. During integration, it is important to ensure that data quality is maintained and data conflicts are resolved.
- Monitoring and reporting: Continuously monitor data quality based on the metrics and dimensions defined in the previous criteria. Create reports and dashboards that continuously monitor data quality.
=> Professional Data Cleansing Services | Ensure Clean & Accurate Data
Technology & Tools
With today’s technology, using the right technology and tools can automate some parts of building a Data quality framework.
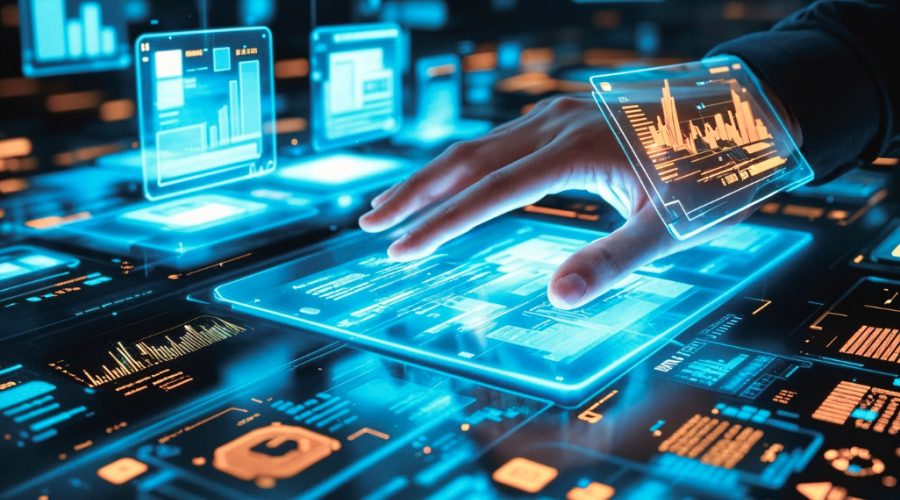
- Data Quality Tools: Are software solutions specifically designed to support data quality management processes such as profiling, validation, cleaning, matching, and data enrichment. Integration: Is the application of technology to integrate data with other existing systems and applications (e.g. databases, data warehouses, CRM platforms).
- Quality Dashboards: Reflect visualization tools that provide a real-time or near-real-time overview of data quality metrics. Help stakeholders quickly assess the state of data quality, identify trends, and make decisions.
=> Top Data Quality Management Tools for Accurate & Clean Data
Organizational Culture and Awareness
This can be understood as the human element in building a framework. This is an important but often overlooked component in creating a data quality framework.
Company and employees need to have awareness of compliance to ensure the data quality. This is about promoting a culture of data use within the company, which reflects the need for all employees to understand the importance of data and their role in maintaining its quality. This could include providing training, establishing communication channels, and encouraging data-conscious behavior. When employees are aware of data quality policies and standards and adhere to them, the organization benefits from more reliable data. Building a strong data quality management framework depends on the participation and commitment of everyone in the company and organization.
Benefits and Challenges of Data Quality Frameworks
Implementing a data quality framework will certainly bring significant benefits to an organization, however, the process also comes with certain challenges that need to be anticipated and addressed.
Benefits of a Data Quality Framework
Improved decision making: With accurate, complete, and timely data, business leaders can make more confident strategic and operational decisions. High-quality data will help dispel uncertainty and hesitation in decision-making among leaders.
- Improved operational efficiency: Consistent data helps streamline business processes, reducing errors and delays. This can lead to significant cost savings and increased productivity.
- Increased compliance: Many industries must follow strict data processes. A data quality framework helps ensure that data meets requirements, avoiding data loss and security incidents.
- Improve customer satisfaction: When customer data is accurate and a company executes personalized campaigns well for customers, it will help improve customer loyalty to the brand.
- Increase revenue: High-quality data can be used to identify new revenue opportunities, optimize marketing campaigns, and improve customer targeting and market expansion.
- Reduce risk: Inaccurate data can lead to poor business decisions, financial problems, and other operational risks. A data quality framework helps identify and mitigate data-related risks.
- Empower data analytics and artificial intelligence: Advanced analytics, machine learning, and AI initiatives rely heavily on high-quality data. A good data quality framework will be a necessary foundation for the success of machine learning and data analytics projects with AI.
- Promoting a data-driven culture: By emphasizing the importance of data quality, a data quality framework helps instill a culture where data is viewed as a valuable asset and is actively managed by members of the organization.
=> Financial Data Quality Management: Best Practices for Accuracy and Integrity
Challenges of a Data Quality Framework
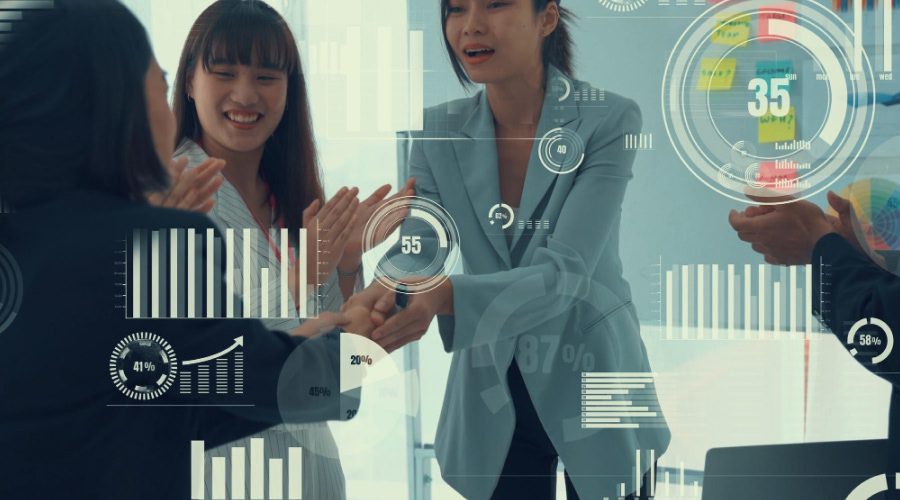
- Lack of leadership buy-in: Without commitment and support from managers, data quality initiatives are unlikely to be implemented systematically.
- Cost and resource constraints: Establishing and maintaining a data quality framework requires investment in technology, processes, and skilled personnel. This can be a barrier for some organizations, especially small and micro businesses.
- Data complexity: Modern organizations often process large volumes of data from many different sources, and if diverse formats and structures are not systematized, creating a framework for the data will be difficult.
- Changing the organizational culture: Shifting to a data quality-first culture requires a change in mindset and behavior across the board. Training and educating employees on the new process is certainly a major challenge.
- Lack of specialized skills: Implementing and managing a data quality framework requires specialized knowledge and skills in data management, and finding and retaining talent with data skills can be difficult in today’s environment.
- Measuring return on investment (ROI): Quantifying the financial benefits of data quality improvements is not straightforward, so companies that insist on ROI will not see the long-term benefits that a data quality framework will bring.
- Sustainability of efforts: Maintaining data quality is not a short-term project but an ongoing process. Maintaining focus and effort over time to ensure sustainable data quality can be a challenge for businesses without a dedicated department.
- Integrating with legacy systems: Many organizations have legacy systems that may not easily integrate with modern data quality tools and processes that today’s tools support.
Comprehensive Strategies for Effective Implementation
A successful data quality framework implementation requires a comprehensive approach and methodology. This is not just about implementing new technology, but also about driving change in the workforce.
Evaluate Your Current Situation
Before you can improve data quality, you need to understand the current state of your data.
- Audit your current data sources: Identify the most important data sources, how data is stored and used.
- Evaluate your data quality: Conduct a data quality assessment using the defined data quality dimensions. This will help identify problematic data files.
- Analyze your existing processes: Review your current data management processes to identify weaknesses, inefficiencies and areas for immediate improvement.
- Talk to stakeholders: Talk to data users across the organization to understand how they are implemented and their needs.
Establish Your Vision for Data Quality
What does the business need to use the data for? What is the vision for the business when it comes to data quality? These are the questions to answer.
- Define the business goals: Be clear about how data quality will impact the overall business goals. For example, improve customer satisfaction by x%, reduce operating costs by x%, or increase compliance with data-driven processes.
- Define data quality metrics (DQ KPIs): The team needs to establish measurable key performance indicators to track changes in data quality.
- Communicate the vision: Ensure that the vision for data quality is clearly communicated and understood by all members of the company.
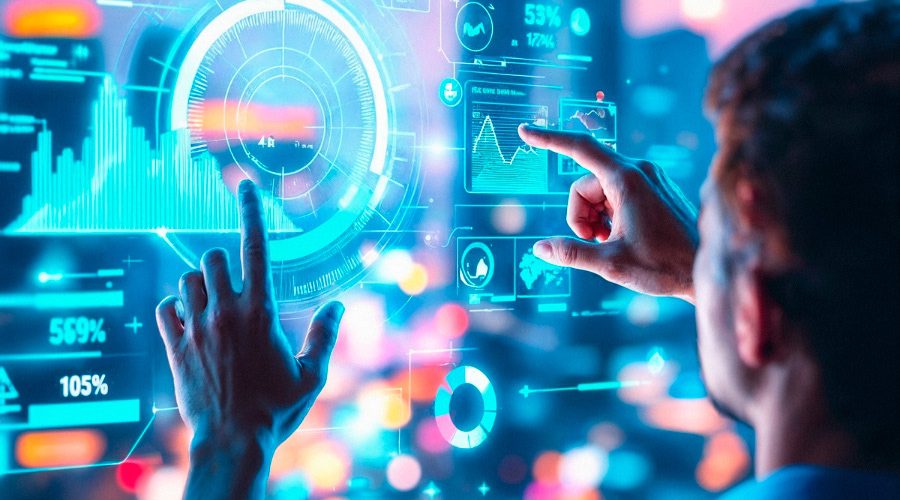
Craft the Framework Architecture
This is where you build the structure of your data quality framework, including the key components you want, but also ensuring the following:
- Define roles and responsibilities: Clearly define who is responsible for different aspects of data quality within the company, including data owners, data stewards, and data quality professionals.
- Establish data policies and standards: Develop clear policies and standards for data creation, management, and use.
- Design a data quality management framework: Outline specific processes for documenting, validating, cleansing, monitoring, and reporting data.
=> Choosing the Right Document Processing Company for Your Business Needs
Automated Document Processing: Enhancing Efficiency and Reducing Errors
Develop the Technical Infrastructure
To save the most time, businesses need to select and deploy the necessary tools and technologies to support the construction of a data quality framework.
- Select data quality tools: Evaluate and select data quality tools that fit the company’s needs and budget.
- Integrate with Existing Systems: Ensure that the selected data quality tools can integrate seamlessly with the existing data infrastructure.
- Set up a data quality dashboard: Create a dashboard to visualize data quality metrics and provide real-time insights to stakeholders.
Create Remediation Processes
Most importantly, the company needs to establish clear processes for addressing data quality issues as things get closer to normal.
- Define an incident reporting process: Establish a mechanism for users to report data quality issues.
- Develop a quick remediation process: Outline the steps to take to investigate, prioritize, and resolve data quality issues.
- Automate remediation processes where possible: Use tools and workflows to automate the correction of common data errors.
Foster Organizational Engagement
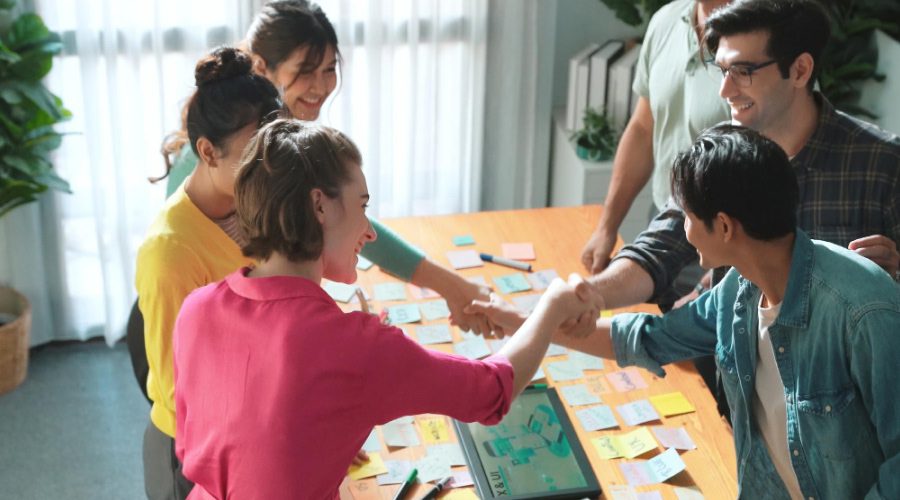
Improving the quality of your business data is everyone’s responsibility. Therefore, the final step is to increase data quality awareness across the entire organization
- Education and awareness: Provide training sessions for employees on the importance of data quality and their role in maintaining it.
- Communicate regularly: Update stakeholders on data quality initiatives, progress, and successes.
- Encourage collaboration: Provide opportunities for departments to collaborate on data quality improvement projects.
- Recognize and reward: Recognize and reward individuals and departments that make significant contributions to improving data quality.
Continuously Improve and Evolve
Data quality is not a short-term goal; it is a continuous journey and requires continuous improvement.
- Monitor and measure regularly: Continuously track data quality metrics and evaluate the effectiveness of the once it is complete.
- Collect feedback: Regularly collect feedback from data users to identify data points that need improvement.
- Adapt to change: Be ready to adjust the data quality framework as business needs, data sources, and technologies change.
- Periodic review: Conduct periodic reviews of the data quality framework to ensure the framework remains relevant and effective over times.
Assess and Convey the Value
Finally, after a period of change effort, see how the data quality has changed, reflected in the results achieved by the business
- Track business impact: Measure the impact of data quality improvements on business outcomes, such as cost reductions, increased revenue, or improved customer satisfaction.
- Improve ROI: Calculate the ROI of the costs you spent and what you get back 6 months to 1 year after the implementation is complete.
Data Quality Framework Examples
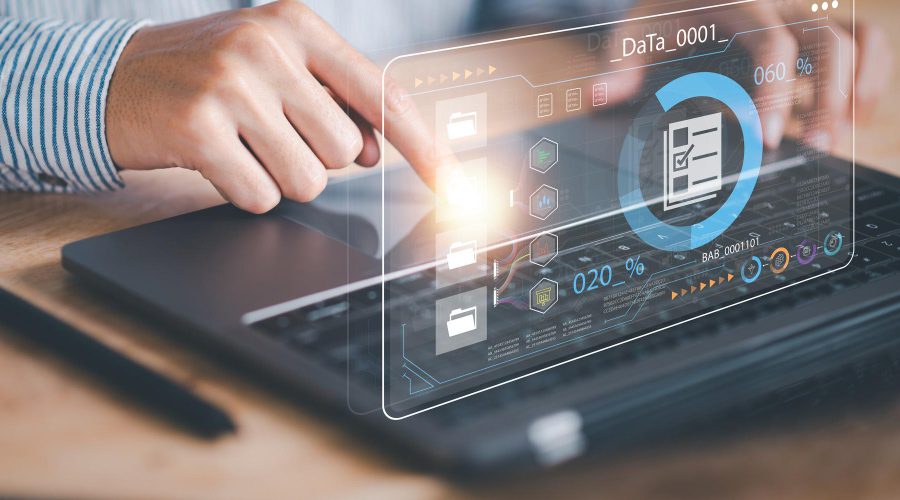
There are many different models and implementation methods that can be adapted to build a data quality framework that fits the specific needs of a company. Below are specific examples of successful data quality frameworks
Data Quality Framework Examples
Typically, companies will take a base from established models or develop successful templates, then customize and combine elements from various sources to fit the needs of the company. The ultimate goal is to create a practical and actionable structure for managing and improving data quality. Below are a series of examples of popular data quality framework templates that can be used as examples for businesses to implement.
DQAF – Data Quality Assessment Framework
DQAF was developed by the International Monetary Fund (IMF) to assess the quality of statistical data. This method is a systematic structure for assessing data quality. Although focused on statistical data, the principles of this template are developed to be applicable in many fields.
- Integrity: Whether the data processing units are equipped with adequate resources and processes to operate appropriately.
- Accuracy and reliability: Whether the data accurately reflects the economic reality at the time it is collected for measurement.
- Relevance: Whether the data is relevant, timely, and consistent.
- Accessibility: Whether the data is clear and easy for users to access.
- Methodology: Whether the methods used to collect and compile the data are reasonable.
TDQM – Total Data Quality Management
TDQM is a data management approach that emphasizes a comprehensive and continuous implementation to improve data quality. TDQM will focus on meeting the needs of customers (data users) and continuous process improvement. The main principles of TDQM include
- Customer focus: Understand and meet customers’ data quality requirements.
- Continuous improvement: Continuously seek to improve data quality processes and outcomes.
- Everyone is responsible: All employees have a role in ensuring data quality.
- Process approach: Data quality management requires one or more specific processes.
- Data-driven decision making: Using data to make business decisions.
DQS – Data Quality Scorecard
A data quality scorecard is a tool for measuring, tracking, and reporting on data quality over time. The methodology will provide an overview of data quality performance based on pre-defined metrics and goals. As a template of the data quality framework, DQS helps:
- Quantify data quality: Assign scores or ratings to different data quality dimensions.
- Track progress: Monitor improvements or deterioration in data quality over time.
- Communicate results: Report data quality to stakeholders in a clear and concise manner.
- Identify data groups that need attention: Highlight groups of data or processes with quality issues.
DQMM – Data Quality Maturity Model
DQMM is a roadmap approach for companies to more easily assess and improve their data quality management capabilities. This approach typically identifies different stages of data evolution, from early (where data quality processes are chaotic) to optimized (where data quality is actively managed and continuously improved). By using DQMM in a data quality framework, organizations can:
- Assess current data maturity: Understand the state of the data in terms of data quality management.
- Identify improvement goals: Set realistic goals to achieve higher levels of maturity.
- Develop an action plan: Outline the steps needed to improve data quality management capabilities.
DDT – Data Downtime
While not a “framework” in the traditional sense, the concept of Data Downtime was popularized by companies like Monte Carlo. DDT refers to periods of time when data is corrupted, inaccurate, missing, or unreliable. Focusing on minimizing DDT can be another approach to building a data quality framework
- Detect issues early: Implement tools and processes to identify data downtime as quickly as possible. Do not let the incident escalate and affect the company’s reputation.
- Inform affected departments: Set up tools to alert end users and departments working with that data about ongoing issues.
- Resolve incidents quickly: Processes need to be in place to quickly investigate and fix the root causes of data downtime.
- Prevent future incidents: Analyze DDT incidents to identify patterns of bad data and take preventative measures in the future.
DDT mitigation ensures that data is always available for use and decision making, which is an equally important goal of any data quality management framework.
4 Stages of a Data Quality Framework
To implement an effective data quality framework, you will need to follow a structured, multi-stage process. Here are four key stages that are common in setting up and operating a data quality management framework:
Define Your Data Workflow
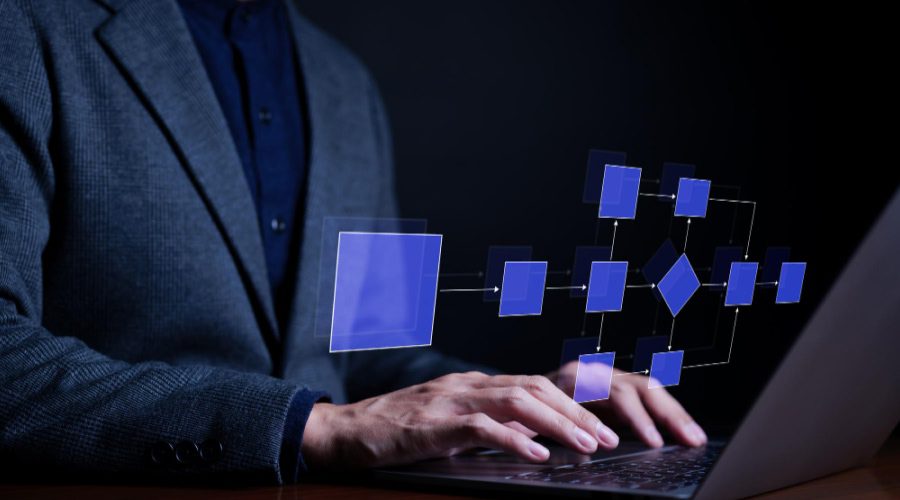
This step focuses on understanding how data operates within the organization.
- Mapping data operations: Identify all data sources, how data is collected, transformed, stored, and used in departmental processes.
- Identify critical data points – CDPs: Not all data is of equal importance. In this step, identify the data that is most important to your core business.
- Understand your customers and their needs: Engage with customers (or end users of data) to understand how they use data, their expectations of data quality, and the challenges they face.
- Assess Data Quality Risks: Analyze potential weaknesses in your data workflow where errors can occur.
Implement a Continuous Improvement Process for Data Quality Rules
Once you have a clear understanding of your data workflow, the next step is to establish and refine rules that define data quality.
- Identify Data Quality Dimensions: Select the most appropriate data quality dimensions (e.g., accuracy, completeness, timeliness, validity, consistency, uniqueness) for important data groups
- Develop Specific Data Quality Rules: For each dimension, create measurable rules that define what “good” data looks like. For example, the ‘Customer Phone Number’ field must contain 10 digits and cannot be blank.
- Implement data validation mechanisms: Integrate these rules into your data workflow so that data can be validated at the right time
- Establish a feedback loop: Create a process to regularly review the effectiveness of the rules set to improve data quality. As needs change or new data issues emerge, these rules may need to be updated or supplemented.
=> The Importance of Address Validation Software for Businesses
Choose Your Infrastructure
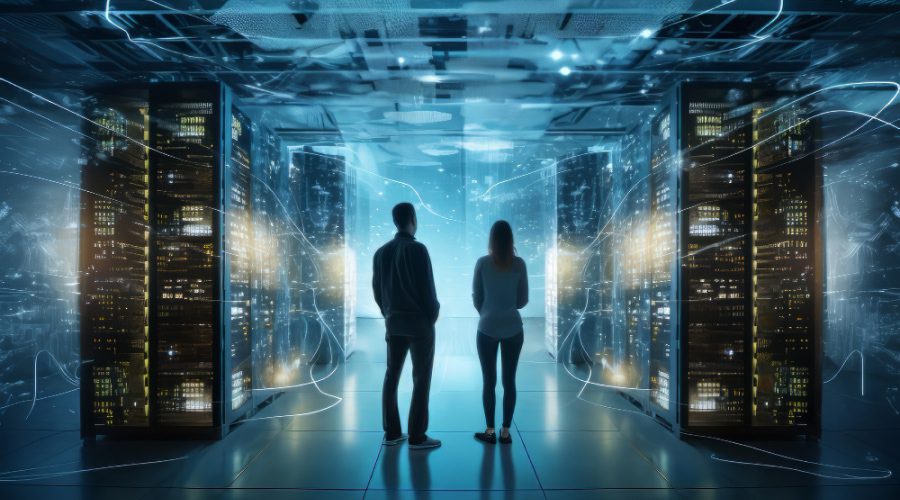
This step will be the step to select and implement the tools and technologies that will support the development of a data quality framework.
- Evaluate existing data quality tools: Determine whether your company’s current tools can meet your data quality management needs or if a completely new investment is needed.
- Select the right tools: Choose data quality tools based on your company’s needs. For example, the ability to data profiling, data cleansing, data monitoring, metadata management, and integration with existing company systems.
- Integrate tools into workflows: Ensure that selected tools are seamlessly integrated into employee data workflows to automate data quality processes.
- Train users on new tools: Provide comprehensive training sessions for departments that will use data quality tools.
=> Essential Data Cleaning Techniques for Accurate Analysis
Evaluate Success Through Data Quality Metrics
The final phase focuses on measuring the effectiveness of the data quality framework and demonstrating value once the business has successfully built it
- Define data quality metrics (DQMs): Develop a clear, measurable set of metrics to track data quality over time.
- Implement monitoring and reporting: Establish processes to regularly monitor DQMs and generate reports for stakeholders.
- Quantify the impact on business performance action: Try to sit back and connect improvements in data quality to tangible business outcomes, such as cost reduction, increased efficiency, or improved customer satisfaction.
- Iterate and refine: Data quality is an ongoing effort. Refine the framework so it stays relevant over time.
Data Quality Framework Tools
To effectively deploy and operate a data quality framework, it will be necessary to have the support of specialized tools. Here are some prominent tools commonly used to build a data quality management framework:
Monte Carlo
Monte Carlo is a platform that helps minimize data downtime. Instead of focusing on manual rules, Monte Carlo uses machine learning technology to automatically monitor data and alert to potential problems that the data may encounter.
- Key features: Automatic error detection, data flow tracking, proactive notifications, root cause analysis of faulty data.
- Data quality framework support: This tool will help identify and resolve data quality issues faster, minimizing the impact of bad data on end users. Monte Carlo Helps Ensure Data Trust
=> See more: Automatic Data Processing Solutions | Streamline Your Workflow
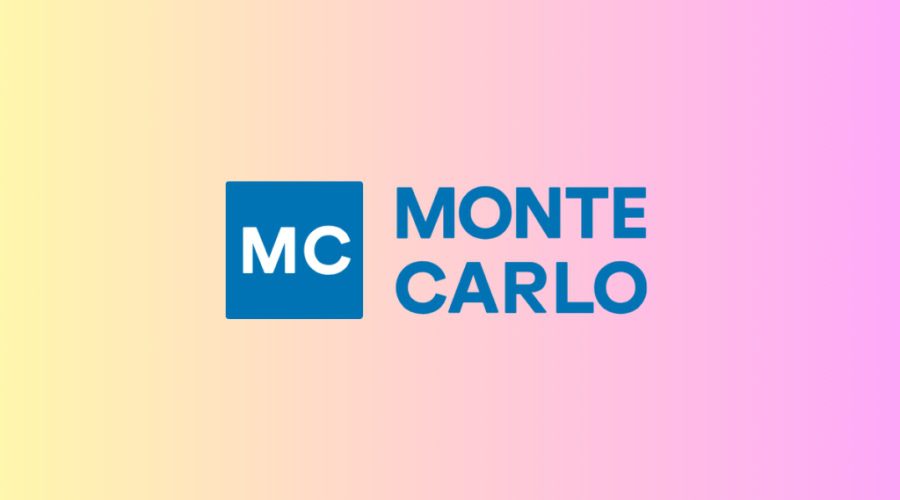
Torch by Acceldata
Torch, a feature of the Acceldata platform, supports features for comprehensive data quality management across modern data systems.
- Key features: Data profiling, rules-based data validation, data reconciliation, continuous data quality monitoring, and metadata management.
- Data quality framework support: Torch helps businesses define, deploy, and enforce data quality rules consistently across disparate data sources.
Great Expectations
Great Expectations is a popular tool for data validation, documentation, and profiling. It helps data professionals visualize what their data looks like and get alerts when those visualizations are not met.
- Key Features: Customizable “Expectations” library, automatic data documentation (Data Docs), batch and stream data validation, integration with various data workflow tools.
- Data quality framework support: This tool offers a more flexible approach to framework implementation, integrating data quality checks directly into where data operates.
OwlDQ
OwlDQ (now Collibra Data Quality & Observability) uses machine learning to automatically detect data quality issues without the need for coding or manual rule sets.
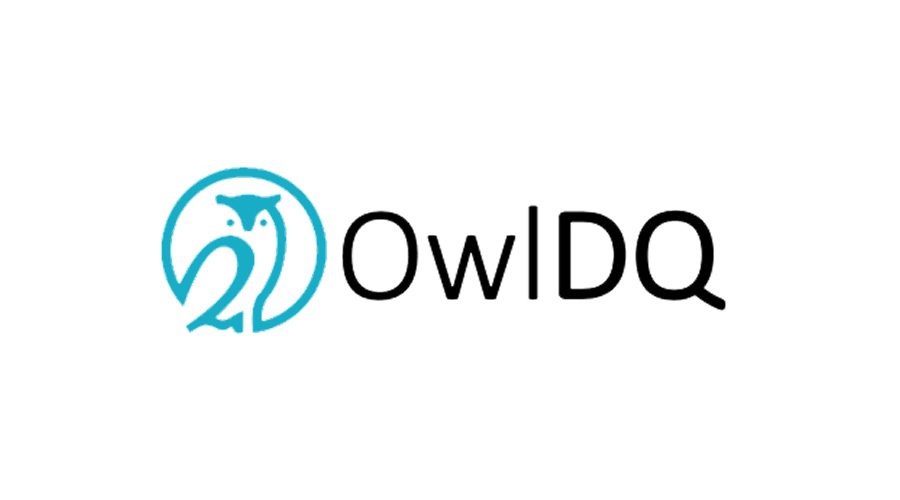
- Key Features: Automated data quality rule suggestions, anomaly detection, intelligent data profiling, continuous monitoring, and an intuitive user interface.
- Data quality framework support: OwlDQ simplifies the process of setting up and maintaining data quality checks by automating the detection of potential threats. The tool can significantly speed up the implementation of a data quality management framework instead of having to do it manually.
Conclusion
In general, establishing a complete data quality framework is a long and ongoing process. Following a phased implementation process and leveraging the right data quality management framework tools will ensure that data is not only accurate and reliable, but also drives better decision making, improves operational efficiency, and provides a competitive advantage. If you are ready to improve your data quality but are still unsure where to start, contact DIGI-TEXX today to learn how our data analytics solutions and expertise can help you build and implement a data quality framework that fits your specific business needs.
=> Read more:
- Reliable Data Entry Service Provider | Accurate & Scalable Solutions
- Data Extraction Automation: Enhancing Efficiency and Accuracy
- The Benefits of Computer Data Processing Services
- Streamlining Operations with Online Data Processing Services
- A Complete Guide to CRM Data Cleansing Services for Effective Management