High-quality financial data quality management is the foundation of successful financial operations. When data is precise, uniform, comprehensive, and current, it enables informed decision-making, supports adherence to regulations, and enhances an organization’s market position.
By adopting robust data management strategies, financial institutions and companies can reduce compliance risks, avoid expensive penalties, and promote a culture of decisions grounded in reliable data.
=> See more: Data Quality Management: Ensuring Accuracy and Reliability
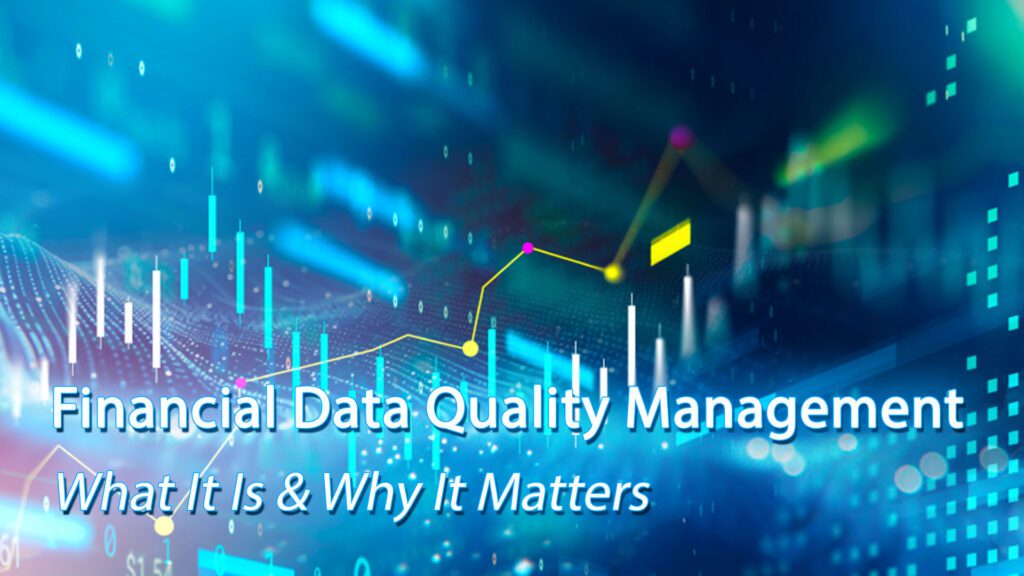
What Is Financial Data Management?
Financial data quality management is the set of policies, processes, and frameworks that an organization uses to ensure its financial data is accurate, reliable, and relevant enough for an educated business decision to be made. Rather than simply resolving issues, it takes a more strategic approach that encompasses data management, oversight, and continual monitoring.
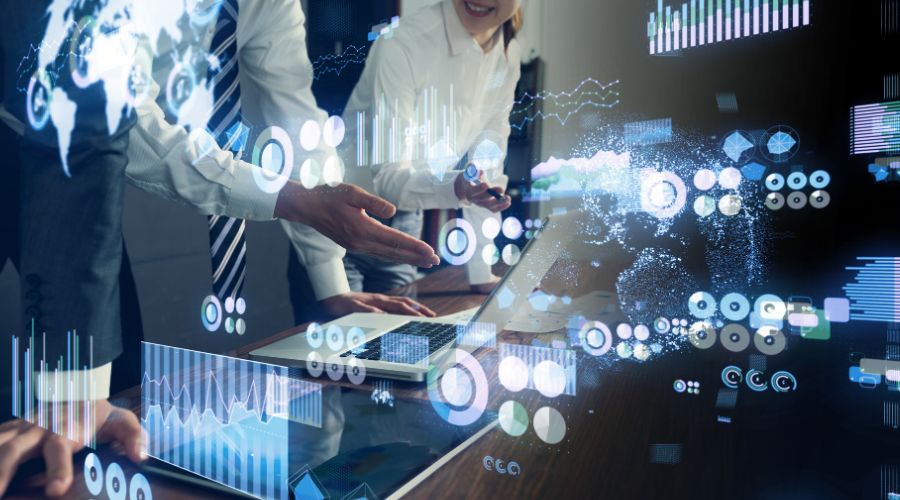
Financial data quality management is the set of policies
What Is Quality In Financial Management?
The quality of data by financial institutions for a given decision-making activity is assessed based on its accuracy, completeness, and timeliness. These factors greatly determine the ability of financial practitioners to make decisions and perform actions. Therefore, data quality can and must be managed within information systems.
As an example, accurate information within financial reports enables better forecasting as well as improved risk management.
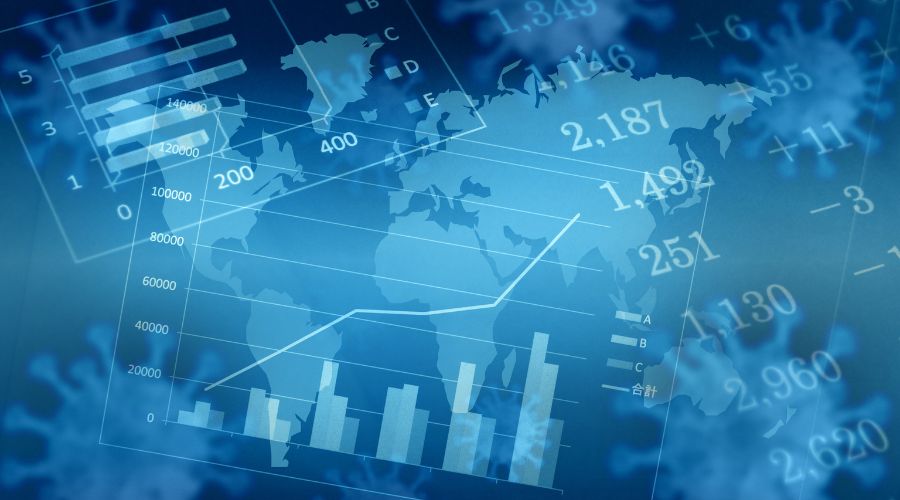
The quality of data by financial institutions for a given decision
What Are The 5 Elements Of Data Quality In Financial Management?
It is important to have a comprehensive approach and system to manage the quality of financial data in an organization correctly so that it meets the requirements of being accurate, reliable, and compliant with the applicable regulations. Here are 5 elements of financial data quality management that you can explore:
Accuracy And Consistency
Decision-making relies heavily on maintaining accurate and consistent financial data within every platform. Any discrepancies concerning transaction logs, clients, or even details in financial statements can pose serious risks, resulting in regulatory violations and losses.
=> Data Accuracy Improvement: Tools and Techniques for Success
Completeness
Data completeness is vital in all administrative processes. Processes such as partially filled customer profiles or incomplete loan applications disrupt risk evaluations and operational workflow. Completing necessary fields should be aligned strategically with the organizational goals.
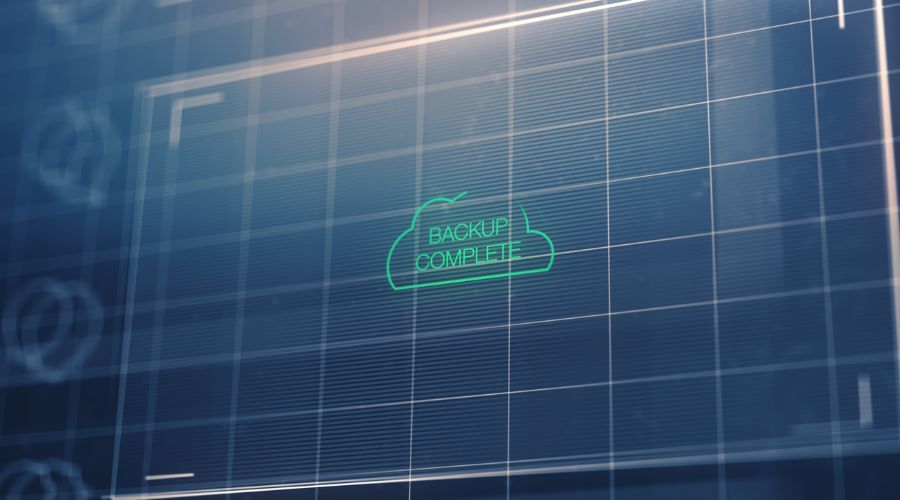
Data completeness is vital in all administrative processes
Timeliness
One of the five elements of data quality management is timeliness. Operations must keep up with industry-leading deadlines, making current information imperative for any organization. Stagnant updates, such as outdated transaction updates and risk analyses, lead the organization to unintentional risk, including non-obvious fraudulent activity or compliance penalties.
Relevance
AML and Basel III regulations highlight the need for robust policy governance and defined precise data formats due to requirements for oversight concerning data accuracy illustrating enhanced oversight concerning non-obvious financial crimes while increasing transparency.
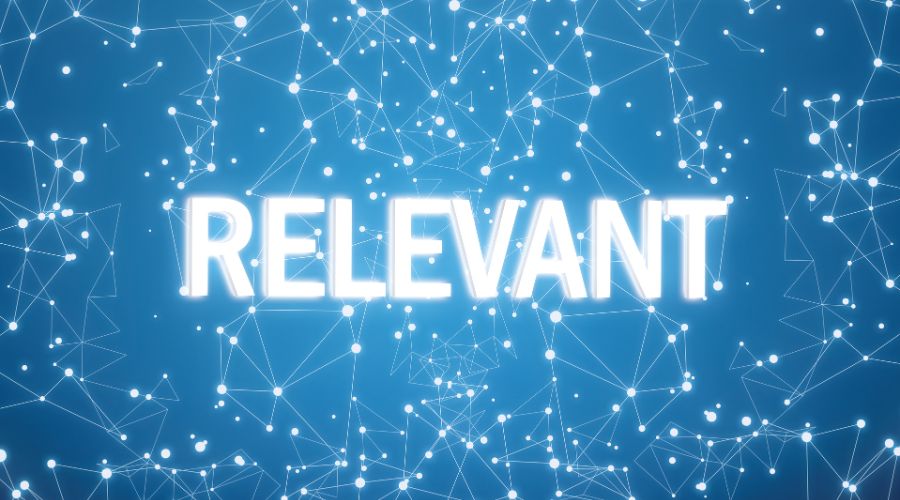
AML and Basel III regulations highlight the need for robust policy
Standardization And Governance
In light of clarity, defined robust policies and standard data formats wielded error reduction. Compliance with regulations like AML (Anti-Money Laundering) and Basel III demands stringent data oversight to prevent financial crimes and enhance transparency.
The Importance Of Managing Financial Data Quality
Financial data quality management is key to producing trustworthy financial reports, meeting regulatory standards, and guiding smart strategic choices. Here is the importance of managing financial data quality that companies should know:
Accurate Financial Reporting
High-quality financial data forms the foundation for dependable financial reporting. Mistakes in the data can lead to flawed financial statements, which might misguide stakeholders and could trigger financial setbacks or legal issues.
Compliance With Regulatory Requirements
Regulatory authorities expect companies to keep precise and thorough financial records. Subpar data quality can result in non-compliance, leading to hefty fines, sanctions, or harm to the organization’s reputation. For example, GDPR enforces strict rules for protecting personal data, and HIPAA requires secure handling of health information. Effective data quality practices, like validation and monitoring, help organizations follow these laws, avoid issues, and build trust.
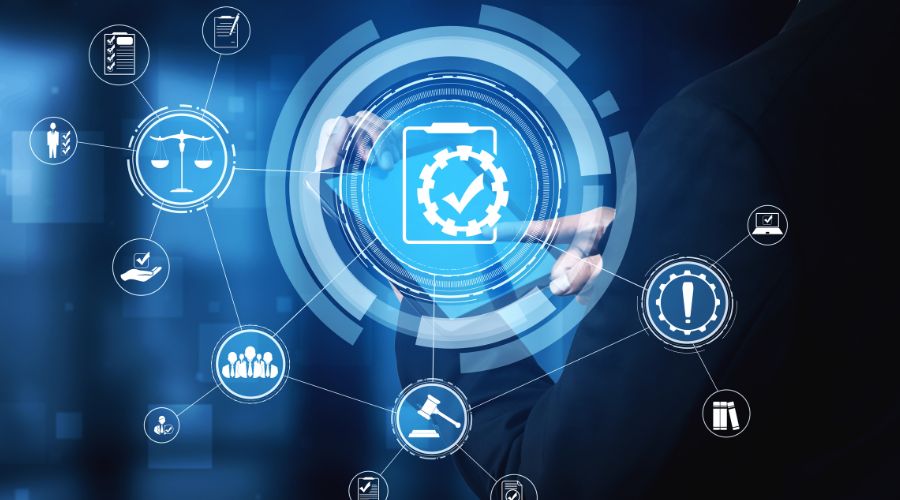
Regulatory authorities expect companies to keep precise records
Strategic Decision-Making
Quality financial data lays the groundwork for effective strategic decision-making. It empowers businesses to spot trends, predict future outcomes, and make well-informed decisions that fuel growth and boost profitability.
Challenges In Financial Data Quality Management
Let’s explore some of the most significant challenges in financial data quality management:
Data Format Variety
Financial institutions handle data from a variety of sources, including transactions, client profiles, and market insights. These arrive in different forms, from structured formats like databases to unstructured ones like emails or scanned documents. Aligning these varied formats to ensure uniformity and precision is a major obstacle, as inconsistencies can spark serious data quality problems.
=> See more:
- Best Practices for Selecting Document Processing Companies.
- How Automated Document Processing Enhances Efficiency and Accuracy.
- Streamline Your Finances with Automated Invoice Processing.
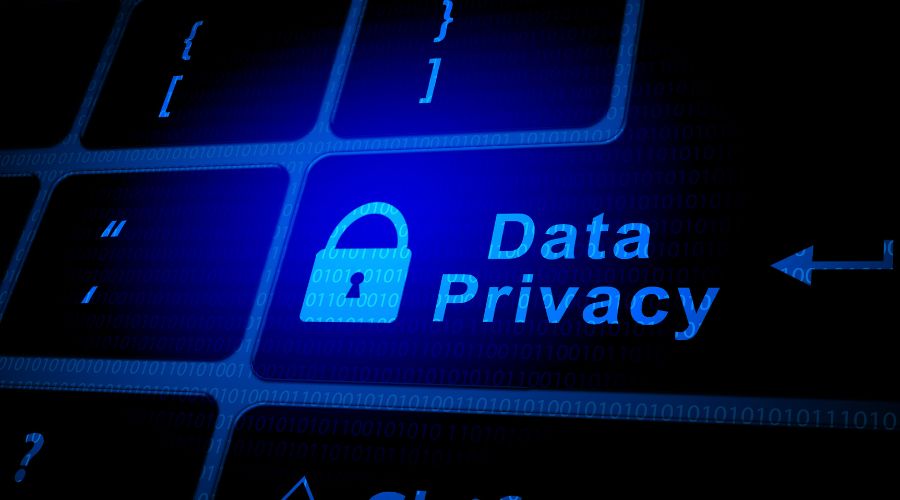
Financial institutions handle data from a variety of sources
Regulatory Compliance
Operating under rigorous regulations like the Basel Accords, GDPR, or regional financial reporting rules, financial institutions must maintain impeccable data quality to stay compliant. Inaccurate or incomplete data can lead to penalties or legal consequences. The challenge lies in keeping data accurate, up-to-date, and comprehensive amid complex datasets and ever-evolving regulatory demands.
Data Discrepancies
When data varies across systems or departments, it can disrupt decision-making processes. For instance, a client’s account information might differ between departments, complicating reconciliation efforts. These mismatches can cause inefficiencies and expose the institution to financial risks.
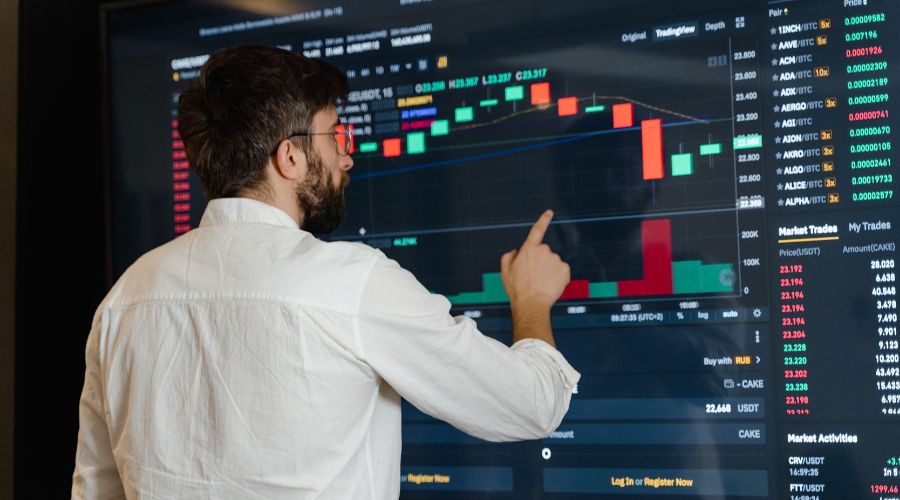
Data that varies across systems or departments can disrupt processes
Risk Management
High-quality data is critical for reliable risk management, enabling accurate evaluations of market shifts, credit risks, and customer patterns. Poor data quality can weaken risk models, leading to flawed analyses and misguided decisions that heighten the institution’s vulnerability to financial losses.
Common Data Quality Issues In Financial Institutions
Data challenges are ubiquitous among financial institutions. Some of them relate to the organization’s operations, compliance workflows and sophisticated decision-making processes. Here are some common issues in financial institutions that DIGI-TEXX wants to provide to you:
Poor Data Quality
A rather endemic problem in many financial institutions is insufficient data quality, consisting of missing, inconsistent, or incorrect data entries. This stems from one or several of the following: legacy systems, manually entered out-of-system data, or poorly integrated automations. Such data inaccuracies can result from non-compliance frameworks, as considering compliance in assessing business processes, determining technological solutions to business problems, severely restricts operational efficiency and performance.
=> How Automated Data Processing Services Improve Accuracy and Speed
Data Validation
Stemming from a lack of adequate control within systems, too much reliance on automation creates problems in the form of falsifying data. Errors go unchecked and propagate throughout multiple systems, known as the Swift Syndrome. Customer-related issues, but complex reporting and strategic calculations, which determine system activities and operating strategies, further complicate systems.
=> How Address Validation Software Improves Customer Data Accuracy
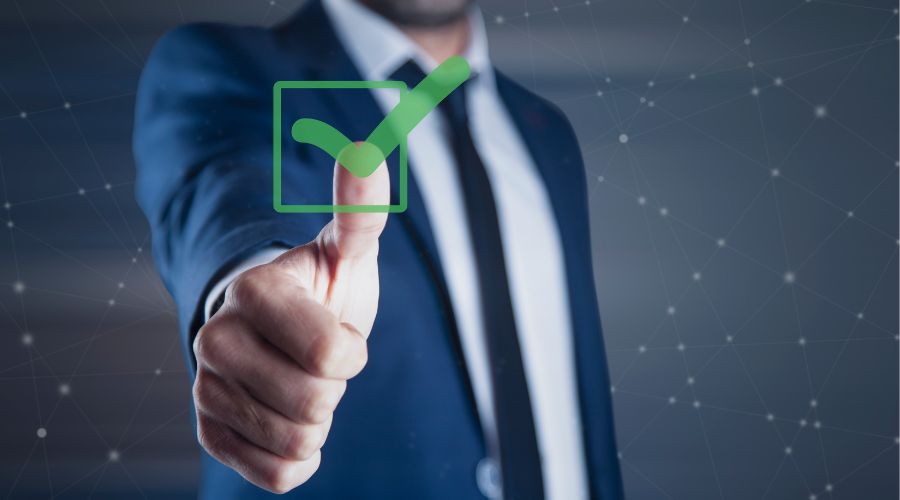
Stemming from a lack of adequate control within systems
Audits And Regulatory Requirements
Tracking and monitoring financial institutions through regulating processes requires constant checking and monitoring. Regardless of the audit cycle, high data quality is an undeniable requirement. Inaccurate reports and inadequate documents can result in failure, sanctions or restrictions. Examples are mishandling controls leading to detection of incomplete, untimely, and inaccurate data. Sturdy data systems around financial reporting are crucial due to unintended consequences, calling for costly, stringent regulations for blatant reporting errors.
Data Governance
Most inadequate reporting frameworks do not determine set policies to enable reliable data entry, processing, storage, and transmission, as well as workflow governance to ensure proper management of information during its lifecycle. Without clear policies, ownership, and procedures, data management becomes chaotic, leading to inconsistent practices and degraded data quality.
=> How a Data Entry Service Provider Can Streamline Your Operations
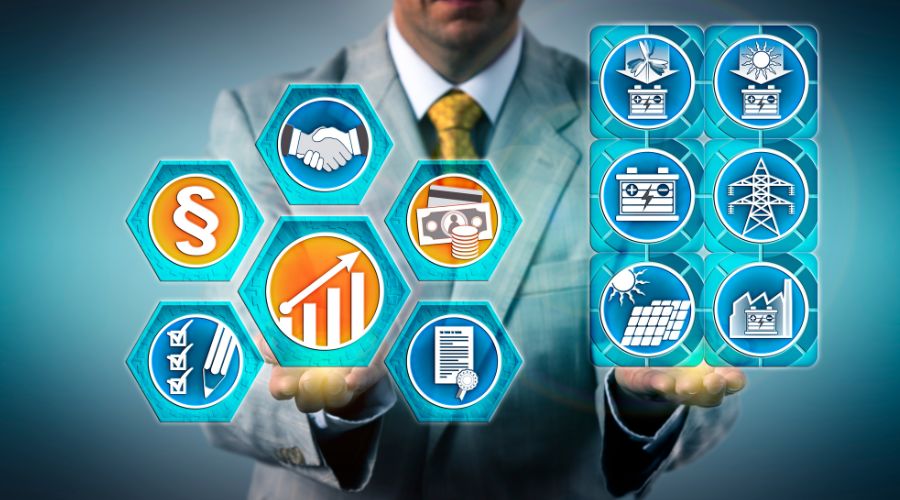
Most inadequate reporting frameworks do not determine set policies
Financial Reporting
Poor data quality can wreak havoc on financial reporting, resulting in inaccurate or incomplete financial statements. This can mislead stakeholders and potentially violate regulatory requirements. To mitigate these risks, institutions must ensure that the data feeding their reporting systems is thoroughly validated and reliable.
Key Steps To Implement Financial Data Quality Management
Obtaining effective and accurate financial data requires a well-defined approach from financial institutions. First, gather everything that’s needed and develop easy and clear steps that will accomplish the goal. Some immediate tasks to implement financial data quality management include:
Define and Understand Data Requirements
Data requirements are the detailed specifications that outline what data an organization needs, including its type, format, source, and quality criteria, to meet specific business or regulatory purposes.
Defining and Understanding Data Requirements involves pinpointing the exact data a financial institution needs for purposes like regulatory reporting, risk analysis, or customer insights. This process entails specifying key data elements, their required formats, and quality standards, while ensuring alignment with business objectives and regulations like GDPR or HIPAA.
This step is critical because it establishes a foundation for consistent, reliable data, prevents costly errors, ensures compliance, and supports confident decision-making.
Assessing Current Data Quality
A reliable data management plan begins with assessing the problem area. In the case of data quality issues, understanding where the problem lies is a multi-faceted process including:
- Increasing processes that diagnose later issues, including parsing, dirty data flags, pattern detection, and pattern verification, such as correcting inconsistencies.
- Checking data consistency, then running a validation engine, will highlight inconsistent, missing, outdated, or duplicate records and even uncredited non-monitored transactions.
- Analyzing acquisition and registry modules for output by using information or record profiling tools.
- Analyzing separate data silos pneumatically through efficient scanners will improve detection of unordered large scale, surrogate, repeat, semantic duplicate and information entries devoid of coherent structures.
Data Cleansing And Standardization
Once an institution develops identification routines, focus can shift to editing uniform criteria. Key improvements should be directed toward:
- Strict measures targeting the removal of duplicates should be taken against known databases.
- Scattering opened files, including naming standards (non methylated) validation dependencies, turned against the opened entire financial silo files should be consistently named or ascribed to use rigidly defined shims.
- Utilizing AI-powered tools to automate data correction and enhancement.
These efforts streamline compliance with complex legal obligations, business dependency graded on risk management, or fraud detection issues.
Or You Can Check Out Our Data Cleansing Services.
=> See more:
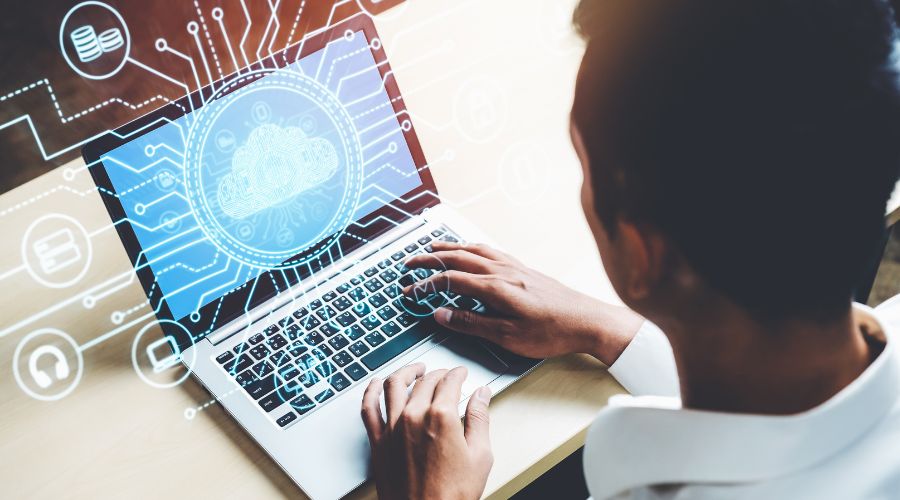
Data cleansing can shift to editing uniform criteria
Continuous Monitoring And Improvement
Expecting ongoing maintenance and having plans to automatically improve the standard also ensures efficiency. Other smart measures may be:
- Intelligent agents are assigned to monitor one-off mitosis and other spontaneous reproduction of errors.
- Data silos automated observability providing active protection and temperature monitoring of finance data will actively alert controllers issuing mandates upon failure tracking.
- Instituting active accountability on data fiduciary work.
- Conducting periodic audits, integrating feedback, and refining processes to ensure lasting data accuracy and integrity.
=> Read more: Top Data Quality Management Tools for Enhanced Data Accuracy
Tips for Managing Financial Data Quality
Given the significance of financial data in an institution, maintaining its high quality requires meticulous supervision and advanced planning. The following suggestions will help guarantee the management of data quality effectively:
Implement A Robust Data Governance Framework
Defining effective data governance policies aimed at the maintenance of high-quality data is fundamental to achieving organizational success. These policies must provide governance at the operational level by allocating specific roles and responsibilities concerning data creation, modification, supervision, and control to every employee in all functions.
Governance aids in solving matters such as duplication of data, records of dead files, erroneous systems, and inconsistencies in logs by enforcing uniform data standards that ensure data integrity.
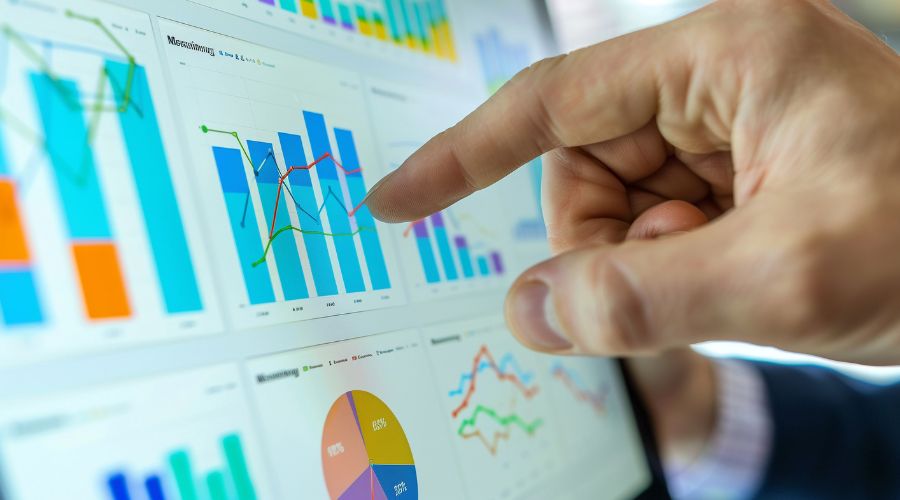
Defining effective data governance is fundamental.l
Use Real-Time Data Management Solutions
As a result of the dynamic nature of financial institutions, formulating and implementing such workflow systems helps perfect data submission as well as updates in the records whenever payment is made. With the use of timely automated updates, a computerized billing system can be incorporated into various financial applications, enabling instant online access and reviews.
Increase Data Observability
Automation provides the opportunity to readily control and manage time-sensitive data, which is in high volumes in financial institutions. Elevation, or the “top-down” approach, is the most common way of handling the huge bulk of compressed and expanded files.
A simple flow meter can provide the necessary parameters and benchmarks required to update such accuracy levels, allowing easy monitoring and reporting within specified parameters and streamlined submission whenever those thresholds are met.
Regularly Audit And Refine Data Management Practices
Regularly perform audits to evaluate the efficiency of your financial data quality management. Through consistent reviews and updates, financial institutions can spot emerging data quality problems early and adjust to evolving regulations, technologies, or internal workflows.
FAQs
What Is Financial Data Quality Management?
Within the umbrella of financial data quality management lies a set of tactics such as processes specifically designed to uphold accuracy, consistency, completeness and credibility of financial information. Data governance, quality assessment, data cleansing, monitoring, and continual improvement are the pillars upon which this management system rests.
How Can Businesses Implement Financial Data Quality Management?
Systematic evaluation of the baseline measurement includes gauging the existing set of standards, cleaning, and applying stringent measures to track data accuracy as well as monitoring data, fostering improvement via feedback, and training through the use of employee training initiatives, which are designed to be self-correcting.
What Tools Are Used In Financial Data Quality Management?
Identifying financial data quality does not rely on one tool but encompasses a wide range, such as compilers that monitor and report on data accuracy, cleaning tools, systems designed for supervising data trends, and predictive analytics through advanced machine learning systems capable of pattern recognition.
Conclusion
Financial data quality management as the foundation of financial operations is now an integral component of modern business practice, not just a simple value add.
Robust regulatory structures help companies avert incurring hefty fines or penalties due to errors in financial reporting. Improving operational efficiency also stems from enhanced data accuracy and reliability provided by ongoing monitoring and routine cleansing of data governance frameworks. For tailored solutions in achieving these goals, explore the innovative data management services offered by DIGI-TEXX.
=> Read more: